The Lesser-Known Differences Between Data Analytics and Data Science
Contents
Data analytics and data science are closely related but they have distinct differences in focus, processes, and goals. While both involve working with data to extract insights and make informed decisions, here are some lesser-known differences between data analytics and data science.
Brief explanation of the importance of Data Science and Data Analytics
Data science and data analytics play crucial roles in today’s data-driven world. They are instrumental in extracting actionable insights from data and fostering innovation across various domains. Their combined impact contributes significantly to the success and competitiveness of modern businesses and organizations.
The important role of Data Science:
Data science enables organizations to build predictive models that forecast future trends and outcomes. This is particularly valuable for proactive decision-making and strategic planning.
By extracting meaningful patterns and insights from large datasets, data science empowers decision-makers to make informed choices based on evidence and analysis rather than intuition alone.
The important of Data Analytics:
Data analytics assesses historical data to review the performance of various aspects of a business, such as marketing campaigns, sales efforts, and operational efficiency.
By analyzing data, organizations can identify inefficiencies in their processes and make data-driven adjustments to streamline operations and improve overall productivity.
Data analytics provides valuable insights into customer behavior, preferences, and demographics. This information is crucial for tailoring marketing strategies and improving customer satisfaction.
What is Data Analytics?
Data analytics is the process of examining and interpreting data sets to extract meaningful insights, draw conclusions, and support decision-making. It involves the use of various techniques and tools to analyze large volumes of data, identify patterns, trends, and relationships, and transform raw data into valuable information. The primary goal of data analytics is to uncover useful information, make informed predictions, and guide business strategies.
The role of Data Analytics
The role of data analytics is multifaceted and plays a crucial part in various aspects of modern organizations. Here are key aspects of the role of data analytics:
- One of the primary roles of data analytics is to provide relevant and timely information to support decision-making processes. By analyzing historical and real-time data, organizations can make informed choices, optimize strategies, and respond effectively to changes in the business environment.
- Data analytics assesses the performance of different aspects of a business, such as marketing campaigns, sales efforts, and operational processes. This evaluation helps organizations understand what works well and where improvements can be made.
- Data analytics helps organizations identify trends, patterns, and correlations within large datasets. Recognizing these insights can lead to strategic advantages, such as anticipating market trends, understanding customer preferences and predicting future outcomes.
Key responsibilities of Data Analysts
Data analysts play a crucial role in organizations. They take responsibility for transforming raw data into actionable insights, supporting decision-makers, and contributing to the data-driven decision-making processes within organizations. Their responsibilities can vary depending on the industry and specific organizational needs but they will still perform key tasks that a data analyst does.
Tasks of Data Analysts
The tasks of data analysts can vary based on the specific needs and priorities of the organization they work for. However, here are some common tasks that data analysts typically perform:
- Gather data from various sources, such as databases, spreadsheets, and external APIs, ensuring data integrity and relevance to the analysis goals.
- Clean and preprocess raw data to remove inconsistencies, handle missing values and format data in a way that is suitable for analysis.
- Conduct exploratory data analysis to understand the characteristics of the data, identify patterns and generate initial insights. This may involve creating visualizations and summary statistics.
- Apply statistical techniques to analyze data, test hypotheses, and derive meaningful conclusions. Statistical analysis helps validate findings and assess the significance of observed patterns.
Emphasize the focus on deriving insights from data
By emphasizing the focus on deriving insights, data analysts contribute directly to the value that data brings to an organization. It’s not just about analyzing data for the sake of analysis; it’s about unlocking actionable knowledge that drives intelligent decision-making and contributes to the overall success of the business.
What is Data Science?
Data science is an interdisciplinary field that involves the use of scientific methods, processes, algorithms, and systems to extract insights and knowledge from structured and unstructured data. It combines expertise from various domains, including statistics, mathematics, computer science and domain-specific knowledge, to analyze and interpret complex data sets.
The role of Data Science
The role of data science is multifaceted and includes a range of activities aimed at a common purpose. Specifically, leveraging advanced techniques and technologies to extract actionable insights from data, supporting decision-making and driving innovation within organizations. Data scientists contribute to solving complex problems and uncovering valuable knowledge that can have a significant impact on business outcomes.
Key responsibilities of data scientists
Data scientists often work in interdisciplinary teams, collaborating with domain experts, data engineers, and business stakeholders. The primary purpose is to ensure that the data science solutions align with organizational goals and contribute to informed decision-making. The responsibilities of data scientists can vary depending on the organization, the industry, and the specific focus of the role.
Tasks of data scientists
The tasks of data scientists encompass a wide range of activities aimed at extracting valuable insights from data. While the specific tasks can vary based on the organization and the nature of the data science project, here are some tasks that data scientists commonly perform:
- Work with stakeholders to clearly define the goals and objectives of the data science project. Understand the business problem or question that the analysis aims to address.
- Gather relevant data from various sources, ensuring that it is comprehensive, representative, and aligned with the project objectives.
- Create new features or variables from existing data to enhance the performance of machine learning models. Feature engineering involves selecting, transforming, and combining features to improve model accuracy.
- Select and implement appropriate machine learning algorithms based on the nature of the problem. Train models on the data and optimize hyperparameters to achieve the best performance.
Mention the broader scope of data science
The broader scope of data science extends beyond traditional data analysis and includes a variety of interdisciplinary elements. The interdisciplinary nature of data science, combining aspects of computer science, statistics, mathematics and domain expertise, contributes to its broad scope. It is a dynamic field that continues to evolve with advancements in technology and the increasing availability of diverse and complex datasets.
The Lesser-Known Differences
Discuss differences that are often overlooked
While data analytics and data science are often used interchangeably, there are subtle differences between the two that are sometimes overlooked. Here are some distinctions:
Data Analytics | Data Science | |
Scope and Goals | Primarily focuses on examining historical data to identify trends, make business decisions, and assess the impact of past events. It is retrospective in nature. | Has a broader scope, encompassing predictive and prescriptive analytics. It involves not only understanding past data but also making predictions and recommending actions to optimize outcomes. |
Techniques and Methods | Often involve descriptive statistics, reporting, and visualization techniques to summarize and interpret historical data. | Encompasses a wider array of techniques, including machine learning, statistical modeling, and advanced analytics. Data scientists may build predictive models to make forecasts and uncover hidden patterns. |
Scale of Data | Typically deals with smaller datasets that can be easily managed using tools like Excel or traditional databases. | Involves handling large and complex datasets, often requiring big data technologies such as Hadoop or Spark for efficient processing. |
Decision-Making Impact | Primarily influences tactical and operational decisions within an organization based on historical data. | Can have a strategic impact, influencing long-term planning, organizational transformation, and innovation. |
Example scenarios where these differences matter
The differences between data analytics and data science can have practical implications in various scenarios, influencing the choice of methods, tools and the expected outcomes. Here are some example scenarios where these differences matter:
Scenario: Demand Forecasting for E-commerce
Data Analytics: An e-commerce company wants to understand historical sales patterns to optimize inventory levels for various products. Data analytics can help analyze past sales data, identify trends, and make decisions based on historical demand.
Data Science: To enhance accuracy in demand forecasting, especially for new products or changing market conditions, data science techniques, including predictive modeling, can be employed. Data science can provide insights into future demand trends, allowing for more proactive inventory management.
Scenario: Predictive Maintenance in Manufacturing
Data Analytics: Analyzing historical equipment failure data to understand patterns and optimize maintenance schedules. Data analytics can help in making decisions based on past maintenance performance.
Data Science: Implementing predictive maintenance using sensors and real-time data. Data science techniques can predict when equipment is likely to fail, allowing for more efficient and proactive maintenance strategies.
Understanding these scenarios helps organizations tailor their approach to data-related initiatives, ensuring that they choose the right methods and tools to meet their specific needs and objectives. Both data analytics and data science have their unique strengths and the choice between them should be based on the nature of the problem at hand and the desired outcomes.
Explain the impact on business decisions
While data analytics focuses on understanding historical data to make informed decisions, data science goes a step further by providing predictive and prescriptive insights that can shape long-term strategy and drive innovation. The impact on business decisions depends on the specific goals and challenges faced by the organization, and the choice between data analytics and data science should align with those objectives.
Conclusion
Understanding these differences can help organizations better define roles, set expectations and leverage the strengths of both data analytics and data science in solving their specific business challenges.
More From Blog
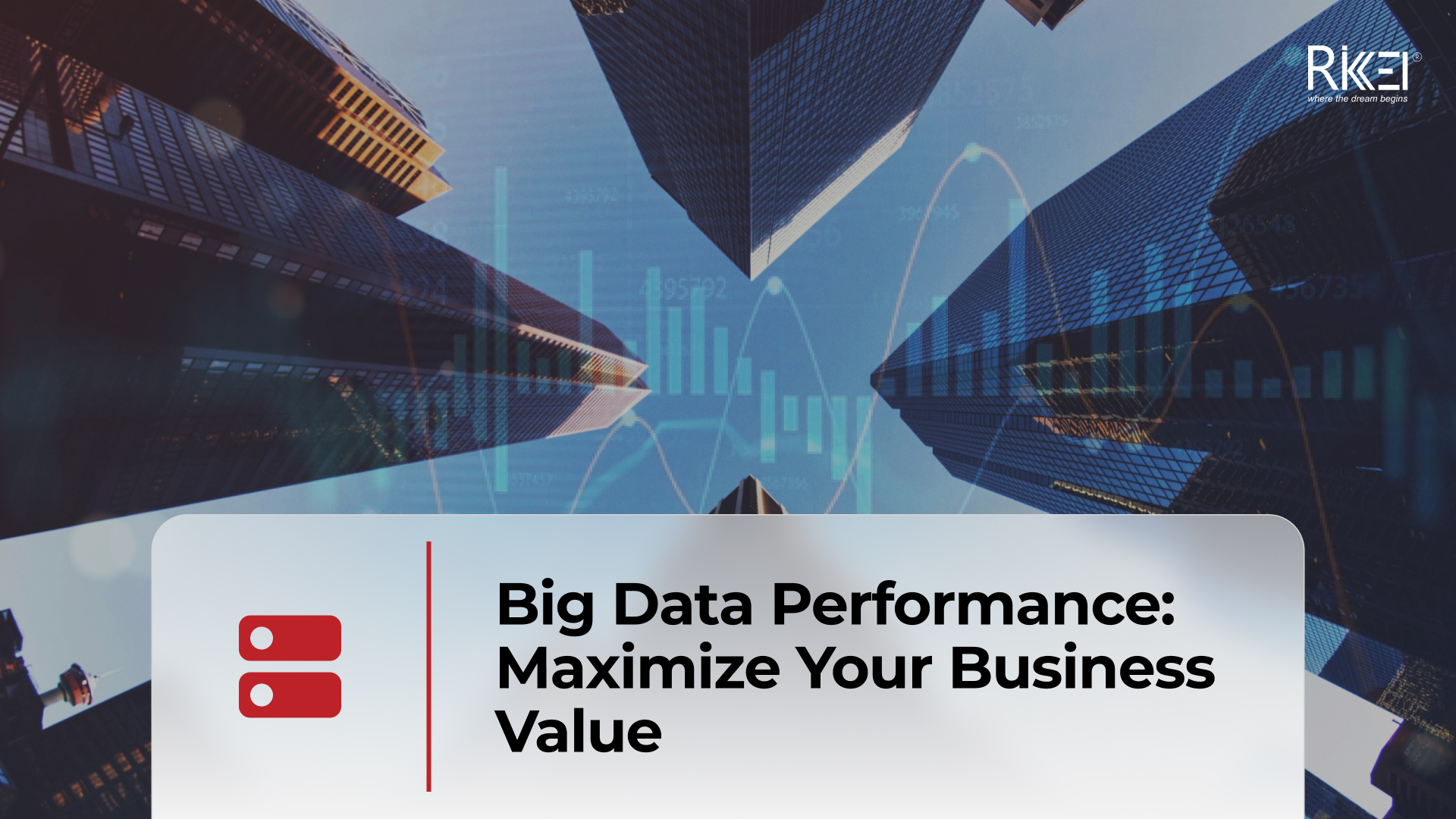
April 4, 2024
Big Data Performance: Maximize Your Business Value
In today’s data-driven world, organizations are constantly generating and collecting immense amounts of data to understand their customers more deeply. This data, often referred to as “big data,” holds immense potential for organizations to seek opportunities and overcome challenges. But accessing and analyzing big data isn’t enough to have proper strategies; organizations must pay attention to […]
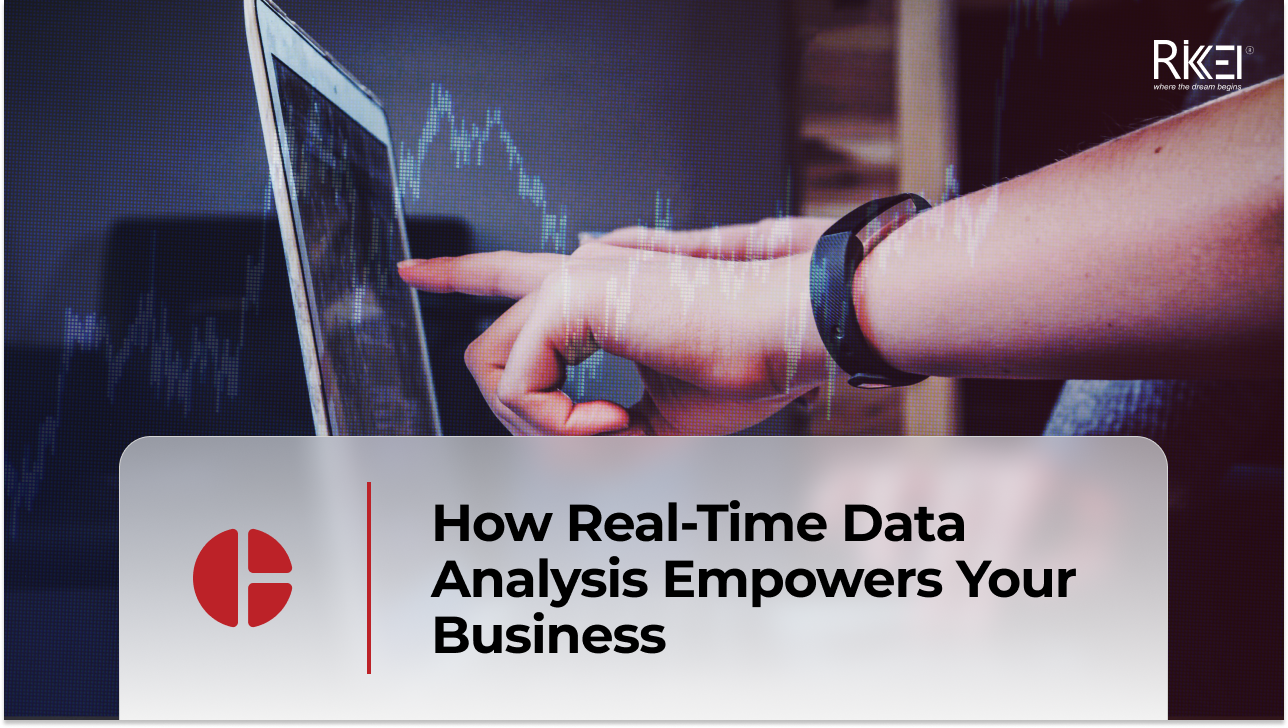
April 4, 2024
How Real-Time Data Analysis Empowers Your Business
In today’s fast-paced business landscape, the ability to quickly make data-driven decisions has become a key differentiator for success. Real-time data analysis, the process of analyzing data as soon as it’s generated, has emerged as a powerful tool to empower business across industries. By leveraging real-time data analysis, organizations can gain timely and actionable insights, […]
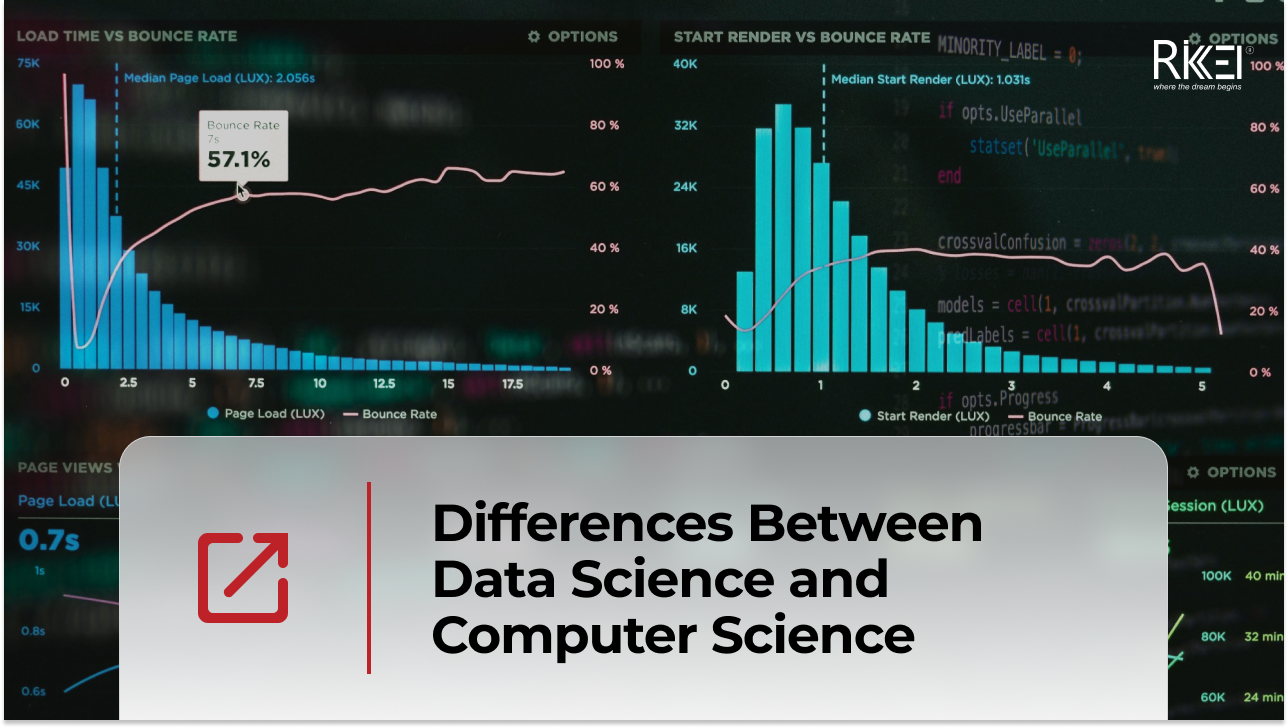
April 4, 2024
Differences Between Data Science and Computer Science
Data Science and Computer Science are distinct fields overlapping in certain areas but have different focuses and objectives. The article below will help you clearly understand the differences and the close connection between the two fields. What is Data Science? Data Science is an interdisciplinary field that combines scientific methods, processes, algorithms, and systems to […]
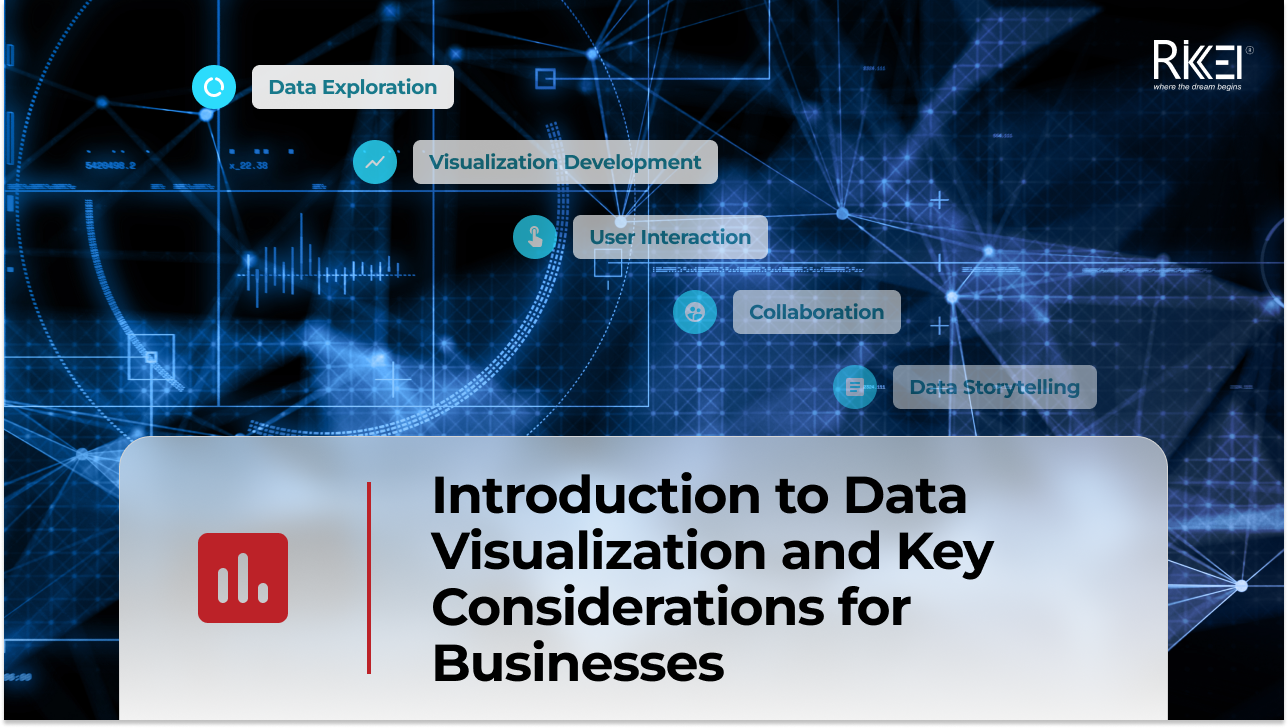
March 28, 2024
Introduction to Data Visualization and Key Considerations for Businesses
In your opinion, what is data visualization? Your main goal is to communicate your recommendations engagingly and effectively, right? To achieve this, let’s immediately explore a method that can represent information with images. What is Data Visualization? Define data visualization and their roles in organizations First, you need to find the answer to the question: […]
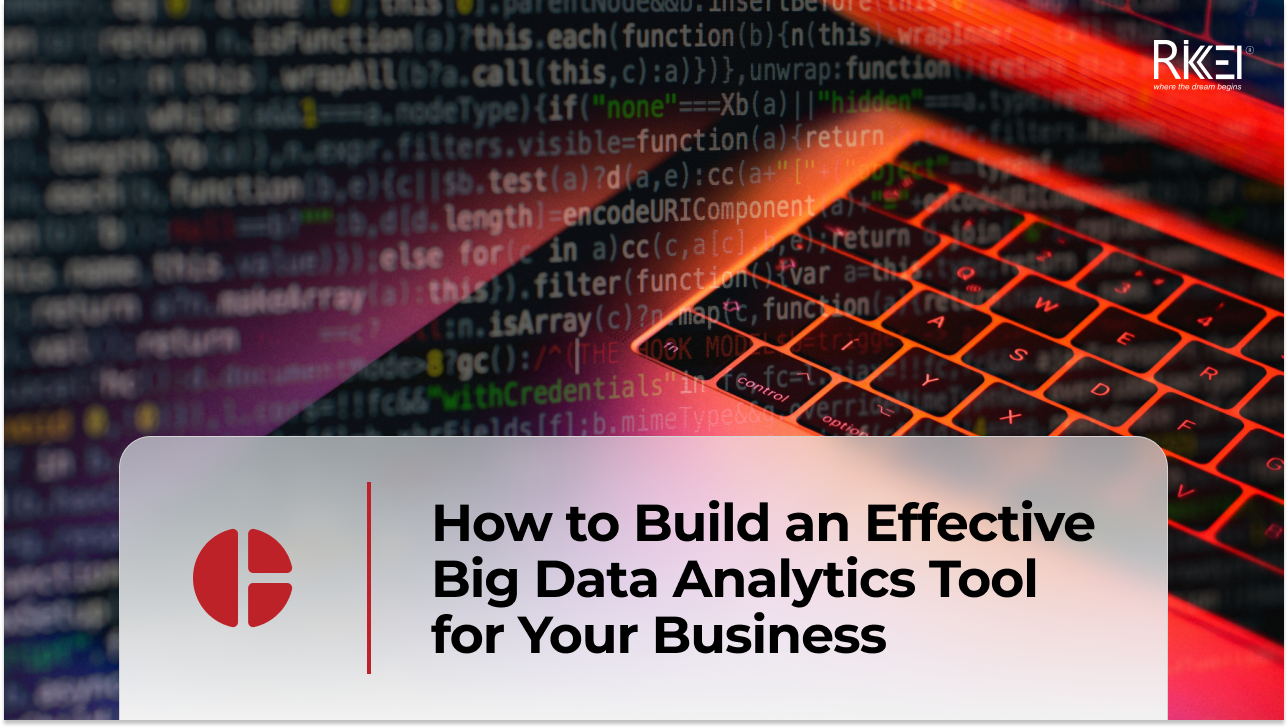
March 21, 2024
How to Build an Effective Big Data Analytics Tool for Your Business
Building an analytics tool for a business brings several significant benefits, especially in today’s business environment where data is becoming larger and more complex. So how to build an effective analysis tool for businesses, follow the article below! Assessing Business Needs Assessing business needs involves understanding the requirements, goals, and challenges of a business or […]
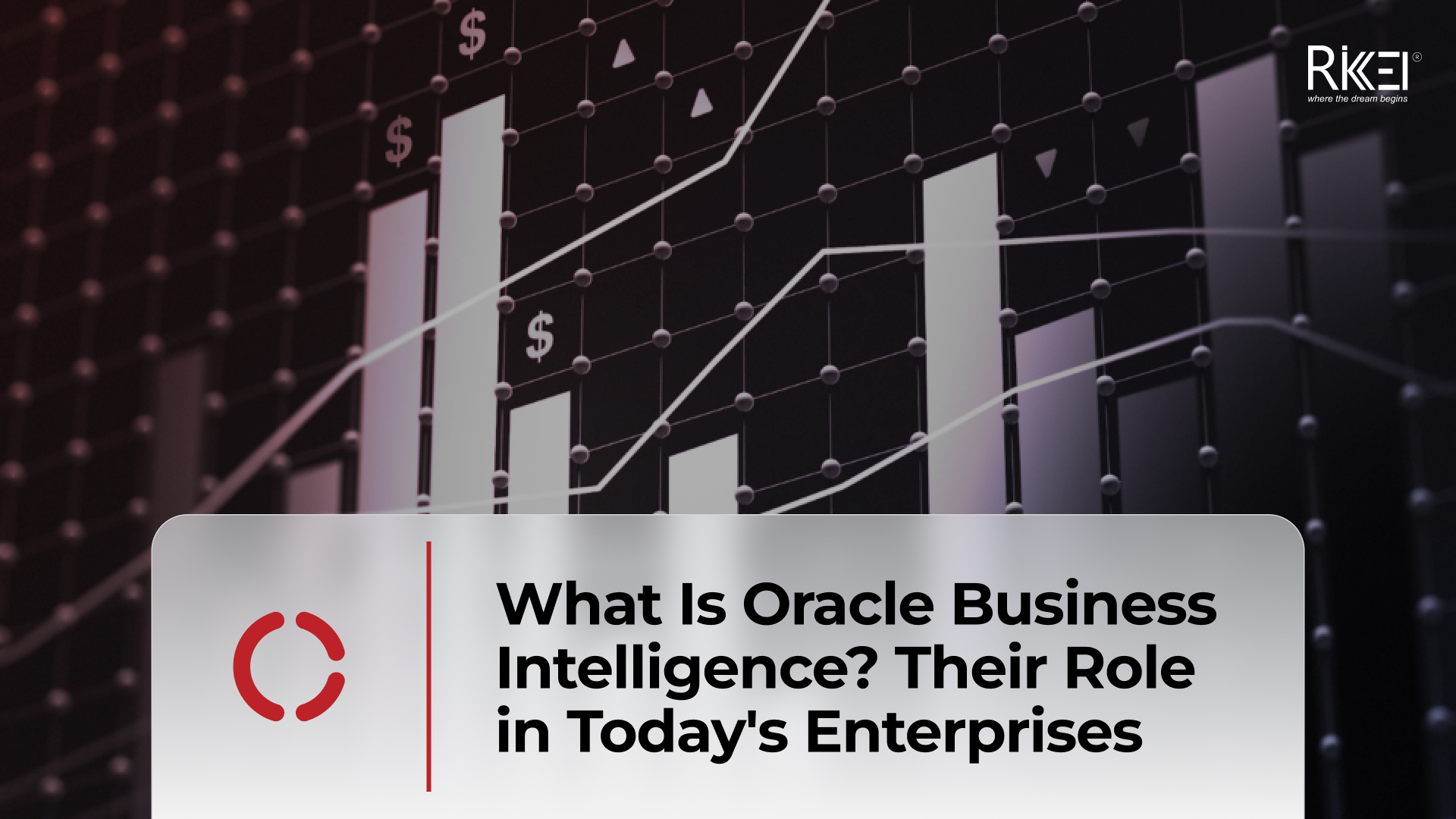
March 14, 2024
What Is Oracle Business Intelligence? Their Role in Today’s Enterprises
Oracle Business Intelligence (BI) refers to a suite of tools, technologies, and applications designed to help organizations collect, analyze and present business data. The primary goal of Oracle BI is to provide actionable insights to support decision-making within an organization. Oracle BI encompasses a range of products that enable users to gather, process and visualize […]