Big Data Performance: Maximize Your Business Value
Contents
In today’s data-driven world, organizations are constantly generating and collecting immense amounts of data to understand their customers more deeply. This data, often referred to as “big data,” holds immense potential for organizations to seek opportunities and overcome challenges. But accessing and analyzing big data isn’t enough to have proper strategies; organizations must pay attention to another critical factor: Big Data Operation Performance (BDOP). BDOP mainly focuses on how efficiently your systems handle the massive datasets involved in big data. Data performance management plays a vital role in managing the organization to meet the business’s objectives. Broadly, big data holds immense potential for businesses in faster decision-making, and ultimately, a competitive edge. This article explores the challenges and benefits of BDOP, its key components, and strategies for implementation to maximize your business value.
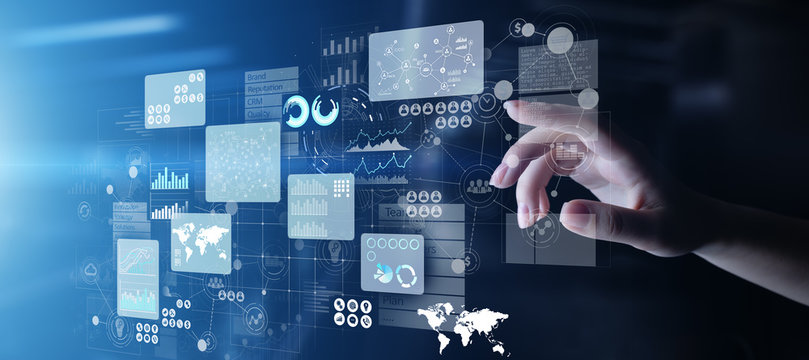
Challenges of Big Data Operation Performance
Big Data offers a treasure trove of insights, but efficiently managing and utilizing it comes with its own set of challenges. The more data you accumulate, the more problems can arise. These challenges include:
- Managing data volume: Big data typically involves large volumes of data across various systems and platforms. The data processing systems need to be constantly scaled to handle ever-increasing volumes. Hence, organizations struggle to get the clean, complete, and consistent data they need for all their systems.
- Data Quality: Big data has various formats and requires diverse tools and expertise to manage efficiently. Moreover, data quality can be a significant challenge in big data environments. The immense variety of data, often originating from disparate sources, can lead to inconsistencies, errors, and missing values.
- Data Real-time Processing: The massive growth in data makes some providers can’t be able to manage to extract value from the huge amount of data. These problems can become more critical and harder to handle as data management and analytics teams attempt to pull in more and different types of data. As a result, slow processing times reduce efficiency and make users have poor experience.
- Performance Bottlenecks: Big data processing also requires significant computing power and resources. It can be challenging for organizations to maintain the data quality, data processing and data query to have a comprehensive visibility with scarcity resources.
By proactively identifying these challenges at first, organizations can develop effective strategies and leverage the promising benefits of the data for innovation and cutting-edge.
Benefits of Effective Big Data Performance Management

In the fast-paced changing technology world, where vast quantities of data are generated constantly, the ability to manage, process, and extract value from this data has become the foundation of organizational success. Data plays a significant role in developing strategic plans so the benefits of implementing robust BDOP practices have never been more pronounced. BDOP contributes significantly to maximizing the return on investment from big data initiatives. Some of the advantages of effective Big Data Performance Management include:
- Enhanced Organization Visibility: By leveraging high-performing Big Data Management, businesses can delve into preferences, purchase behaviors, and even the subconscious desires of their customers through the transformation of databases. So BDOP allows for segmenting customers based on detailed insights. Furthermore, these data can forecast the emerging trends, allowing them to tailor the strategies to position their product services, and messages to specific audience or market.
- Faster Decision-Making: Big Data analytics utilizes advanced algorithms and distributed processing techniques to analyze amounts of data at high speed. This allows businesses to react to both opportunities and challenges in real-time. Hence it drives immense potential by analyzing insights and making better decisions about pricing strategies, customer segmentation, and resource allocation.
- Improved Accurate Insights: Efficient BDOP enables consistent data management practices across the organization. By prioritizing data cleaning and validation, Big Data Performance Management identifies and addresses problems in data volume that could lead to unreliable insights.
- Increased Efficiency and Productivity: Big Data Performance Management helps identify bottlenecks and inefficiencies in data processing. With the clear picture provided by BDOP, businesses can make decisions and develop data-driven strategies for marketing, product development, and overall business growth.
Through ensured data quality measures, optimized processing efficiency, and generation of valuable insights, BDOP transforms businesses into data-driven decision-makers, enhances operational efficiency, and unlocks the full potential return on investment from big data.
Key Components of Big Data Performance Management
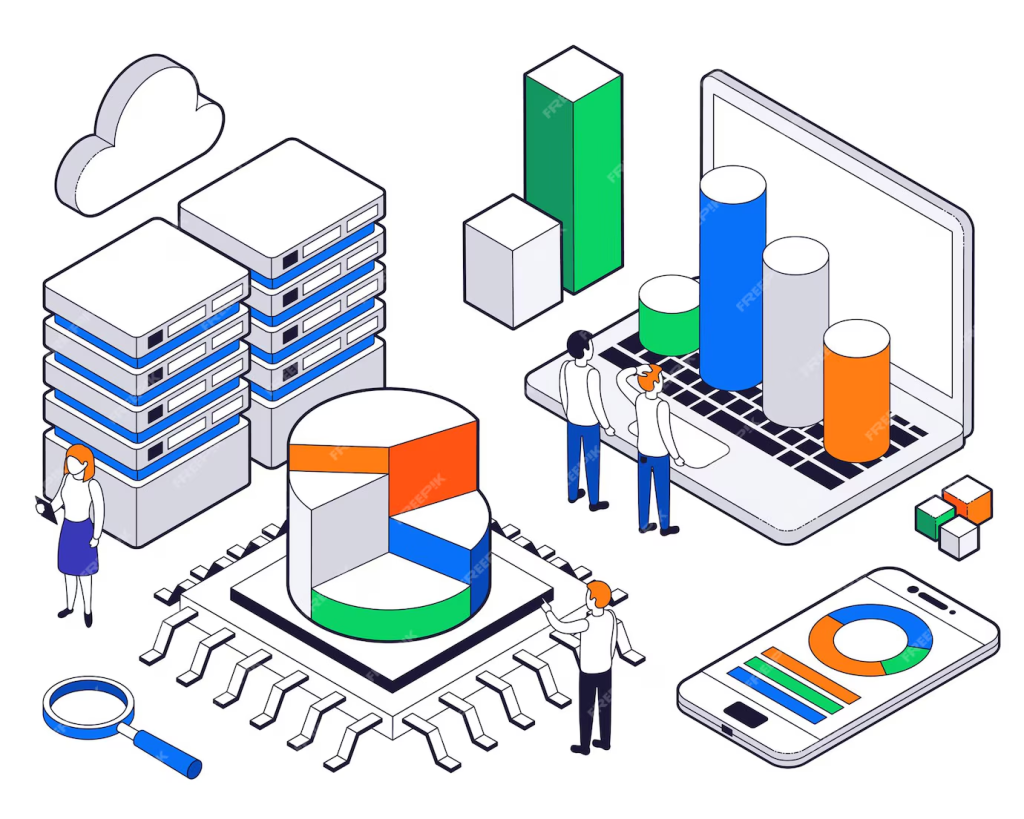
Big Data Performance Monitoring:
Big Data Performance Monitoring (BDPM) provides us critical insights into the health and performance of your data infrastructure. By tracking key performance indicators (KPIs), you can identify bottlenecks, certify data quality, and leverage the hidden value to create a competitive edge.
By monitoring Big Data Performance frequently, organizations can:
- Optimize Performance: By identifying bottlenecks, inefficient queries, and underutilized resources, organizations can optimize data processing tasks, leading to faster and more efficient results.
- Scalability and Elasticity: Assess the system’s ability to scale up or down in response to varying loads, ensuring that resources are allocated efficiently and cost-effectively.
- Data Quality and Integrity: This ensures the data used for analytics and decision-making is accurate, complete, and trustworthy, leading to more reliable outcomes.
BDPM mainly focuses on three areas through KPI monitoring:
- Processing speed: Processing speed in Big data performance monitoring refers to the measure of how quickly a big data system can process, analyze, and deliver insights from large volumes of data. Processing speed is paramount because it determines how rapidly data can be turned into timely insights, directly improving decision-making and related to the rate at which your big data system can complete tasks. By measuring processing times for specific tasks, we can identify risks and optimize performance through better algorithms or increased resource allocation. Hence it provides a comprehensive view of overall processing efficiency. Several monitoring tools designed for big data can help you track how long tasks take, how much data is processed, and other performance measures for example: Application Performance Management (APM) Tools, Big Data Specific Tools and Custom Monitoring Scripts.
- Resource utilization: Resource utilization refers to the percentage of a system’s computing power (CPU, memory, storage, network) that is actively being used at a certain time. The purpose of measuring resource utilization is to identify performance bottlenecks and optimize resource usage to improve the application’s overall performance. By identifying peaks and dips in usage, we can understand how resources are being allocated. It also helps determine if additional resources are needed or if resource allocation can be optimized. Also, it helps an application can better handle higher user loads and scale to meet future needs without requiring additional resources. There are various tools and techniques available to monitor resource utilization, for example, Cloud Monitoring Tools, Operating System Tools and Big Data Monitoring Tools.
- Data quality: Data quality monitoring is the practice of measuring and reporting changes in data quality dimensions and concerns. Well-performance data is the foundation of any successful big data initiative. As organizations increasingly rely on vast volumes of data from diverse sources, ensuring high data quality becomes vital for operational efficiency, strategic planning, and competitive advantage. Regularly review the collected data metrics to pinpoint potential performance bottlenecks or degradation in data operations.
It also can set up alerts specifically for data metrics to identify missing data or error data and collect essential data points that directly impact how well your system handles information. Thus, consistent and reliable data improves operational processes by reducing errors and uncertainties. Some common techniques and tools available to improve data quality include Data auditing, Data cleansing, Data quality rules, Data performance testing, Real-time data monitoring, and more.
Big Data Performance Testing:
Big Data Performance Testing acts as a safety net, making sure your data infrastructure is well-equipped to handle real-world demands.
By addressing these potential performance issues before they impact operations, we can:
- Avoid Performance Issues: Identify and address bottlenecks early on to prevent making failure decisions and enhance seamless experience for users.
- Strategic Resource Planning: Testing provides valuable insights into resource needs. It helps you determine if your current setup is sufficient or if you need to invest in additional resources to meet the demands of your anticipated workloads. It also provides you with an opportunity to assess the effectiveness of your current resource allocation and identify opportunities for optimization based on projected workloads.
- Ensure Data Consistency: By examining data workflows, we uncover potential issues that might lead to data corruption or inconsistencies during periods of high activity, safeguarding the integrity of your data.
Big Data Performance Testing includes these areas:
- Performance Testing: Performance testing is a testing method that evaluates how the system behaves and performs. Software performance testing examines responsiveness, stability, scalability, reliability, speed, and resource usage of your software and infrastructure. After running performance tests, you can breakdown different KPIs and identify bottlenecks and errors. Performance testing uses a variety of methods because it is a superset of other testing techniques, some effective and popular including Load testing, Endurance testing, Volume testing, Scalability testing, Spike testing and Stress testing.
- Load Testing: Load testing is a type of performance test simulating real-world conditions to evaluate how the application responds to varying levels of user activity. Load Testing makes sure that the application can handle the anticipated level of usage and to find any performance-related problems that might occur under typical operating circumstance There are a few types of testing methods including Volume Testing and Scalability testing.
- Stress Testing: Stress Testing is a testing performance pushing the system beyond its boundaries to identify potential bottlenecks and failure points. The purpose of stress testing is to find any performance-related problems that might appear under difficult circumstances and to make sure the application can withstand sudden increases in traffic. Some common techniques for Stress Testing include Spike testing, Volume testing and Configuration testing.
At its core, Big Data Performance Management priorities on maximizing the value derived from big data by enhancing the speed, accuracy, and reliability of data processing and analysis functions. Overall, Big Data Performance Management is a strategic asset, and it acts as a powerful driver of organizational success when implemented effectively.
Implementing a Big Data Performance Management Strategy
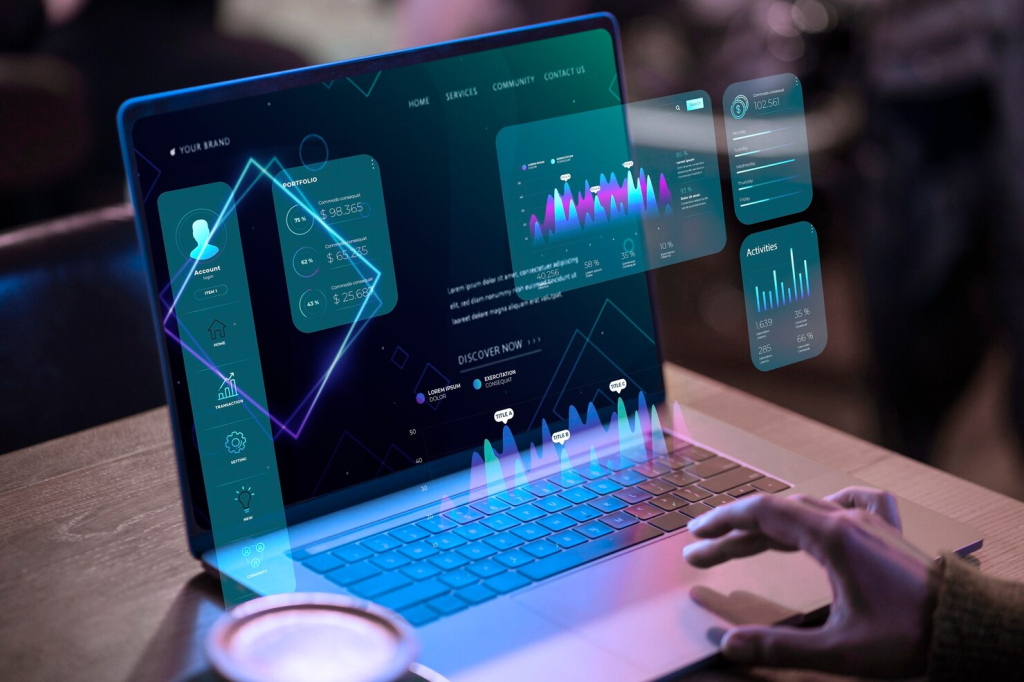
BDOP can bring various potentials for organization, but a well-performance Big Data Performance Management strategy is the key to unlocking its true worth. To implementing a Big Data Performance Management Strategy, business can take these key steps:
Define goals and Key Performance Indicators (KPIs): The root of your BDPM goals depends on your organization’s overall business objectives. Setting certain objectives for big data use and each BDPM goal, specifying measurable KPIs. In turn, faster processing, lower resource costs, and better data quality are the outcomes of this strategic approach.
Choose the right tools and technologies: Optimizing your BDPM strategy requires a careful selection of tools and technologies that not only align with your operational needs but also enhance the efficiency of your data workflows. This strategy is pivotal in navigating the complexities of big data environments and ensuring that your data processing is both effective and efficient. Especially, optimize query performance, ensure that your data model is well-suited for your workload and regularly monitor and tune the system are key design strategies to overcome these challenges. Choosing the right model also depends on the specific type of queries you need to perform most frequently.
Invest in your team: Humans still play a crucial role in ensuring data accuracy because there are issues that require a deep understanding of how the human world works. Additionally, behind a BDPM strategy is always a proficient team that can collaborate the quality data with broader business context.
Effective Big Data Performance Management requires strategic planning and a commitment for continuous improvement. This process ensures your big data systems are optimized for both current and future demands. As a result, it paves the way for data-driven insights that drive better business outcomes.
Conclusion
In today’s data-centric world, strategically managing Big Data Operation Performance is essential for extracting value from your information and achieving significant business success. Additionally, the journey toward maximizing business value through Big Data is continuous, requiring ongoing evaluation, adaptation, and optimization of data management practices to navigate through data challenges. Effective Big Data Performance Management not only enhances operational efficiencies and reduces costs but also unlocks innovative approaches for long-term growth, exact customer targeting, and timely decision-making. Furthermore, to harness the full potential of their data, prioritization is key for organizations, along with strategic investments in the right tools, technologies, and skilled personnel. To sum it up, investing in big data performance is an investment in the future of your business. Embracing effective BDOP, organizations can unleash the transformative power of big data and achieve significant business success.
More From Blog
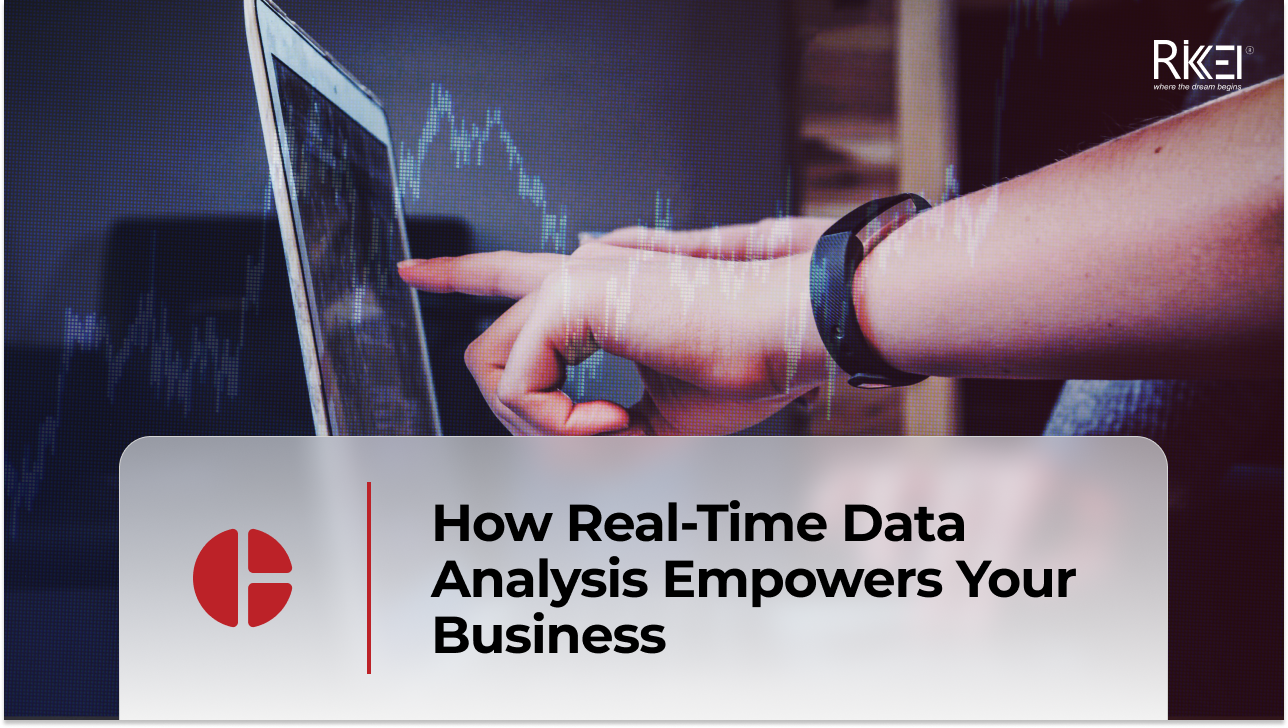
April 4, 2024
How Real-Time Data Analysis Empowers Your Business
In today’s fast-paced business landscape, the ability to quickly make data-driven decisions has become a key differentiator for success. Real-time data analysis, the process of analyzing data as soon as it’s generated, has emerged as a powerful tool to empower business across industries. By leveraging real-time data analysis, organizations can gain timely and actionable insights, […]
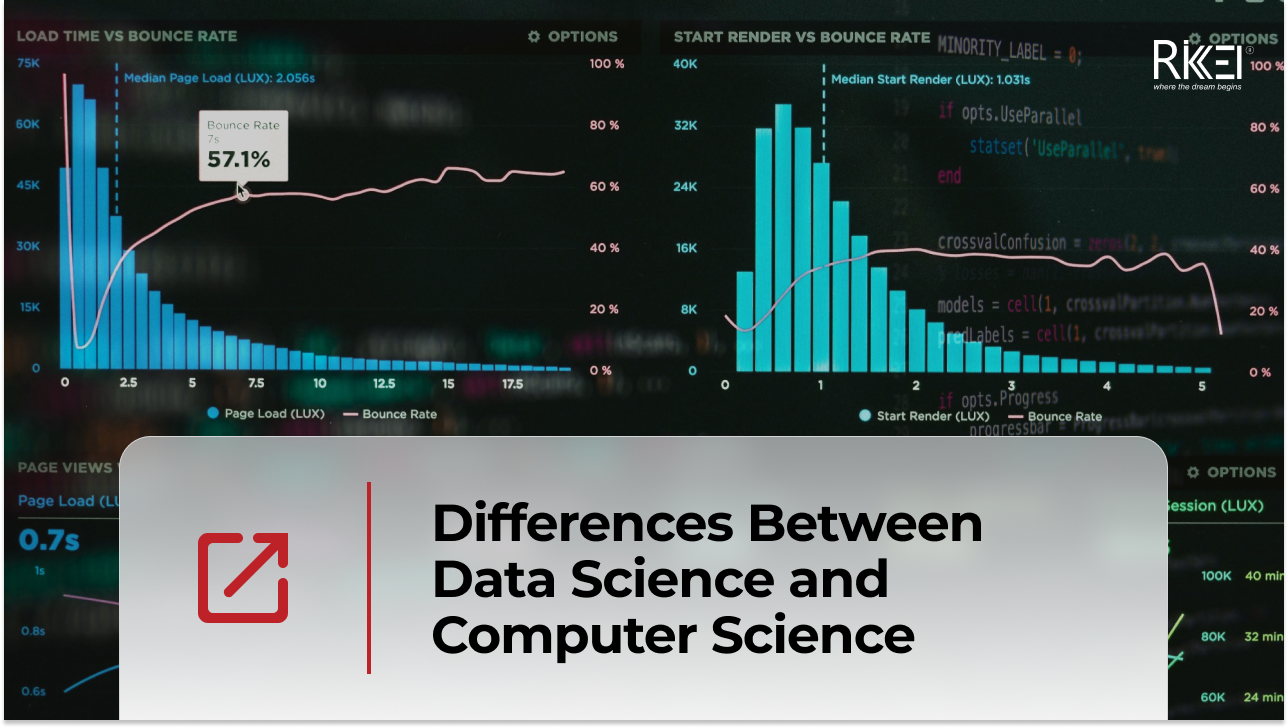
April 4, 2024
Differences Between Data Science and Computer Science
Data Science and Computer Science are distinct fields overlapping in certain areas but have different focuses and objectives. The article below will help you clearly understand the differences and the close connection between the two fields. What is Data Science? Data Science is an interdisciplinary field that combines scientific methods, processes, algorithms, and systems to […]
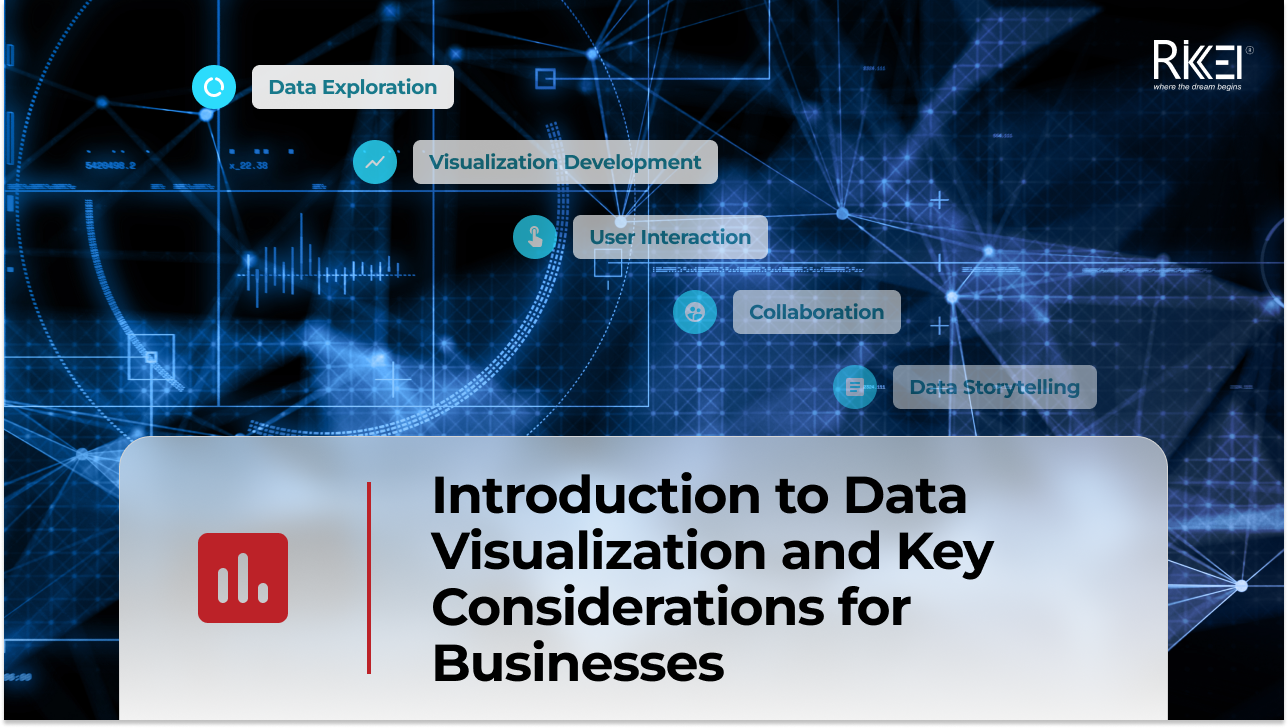
March 28, 2024
Introduction to Data Visualization and Key Considerations for Businesses
In your opinion, what is data visualization? Your main goal is to communicate your recommendations engagingly and effectively, right? To achieve this, let’s immediately explore a method that can represent information with images. What is Data Visualization? Define data visualization and their roles in organizations First, you need to find the answer to the question: […]
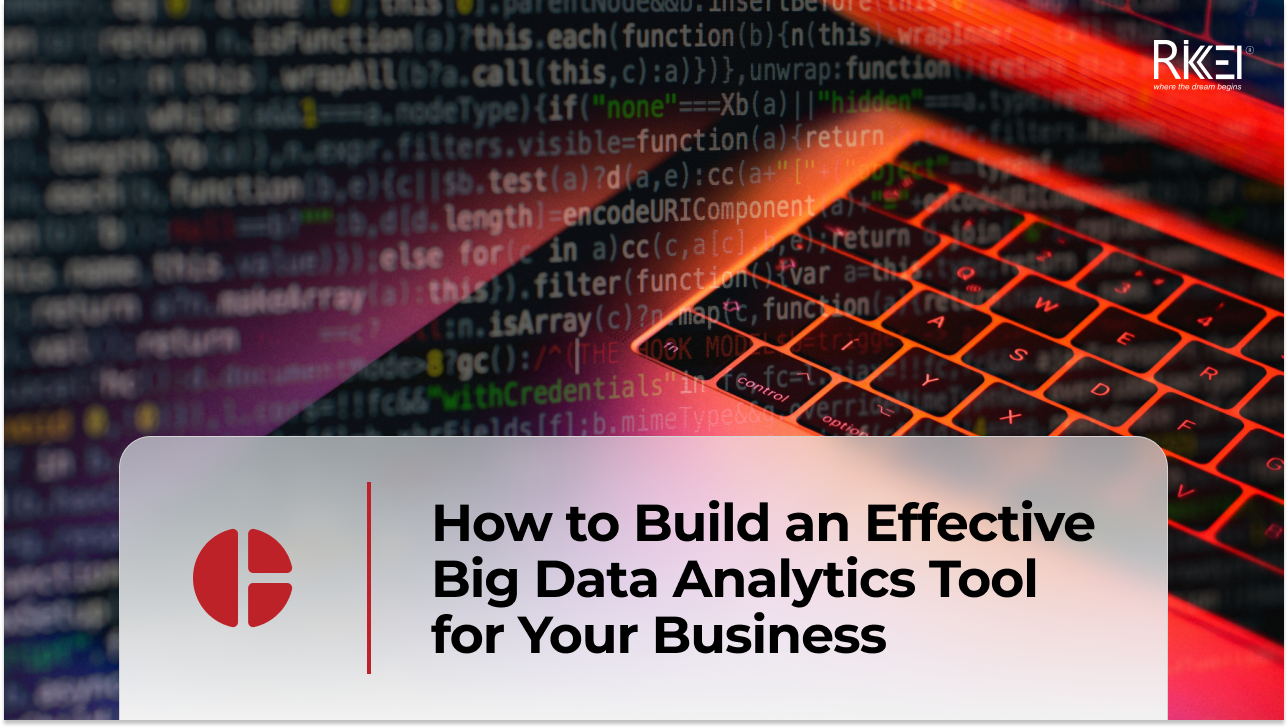
March 21, 2024
How to Build an Effective Big Data Analytics Tool for Your Business
Building an analytics tool for a business brings several significant benefits, especially in today’s business environment where data is becoming larger and more complex. So how to build an effective analysis tool for businesses, follow the article below! Assessing Business Needs Assessing business needs involves understanding the requirements, goals, and challenges of a business or […]
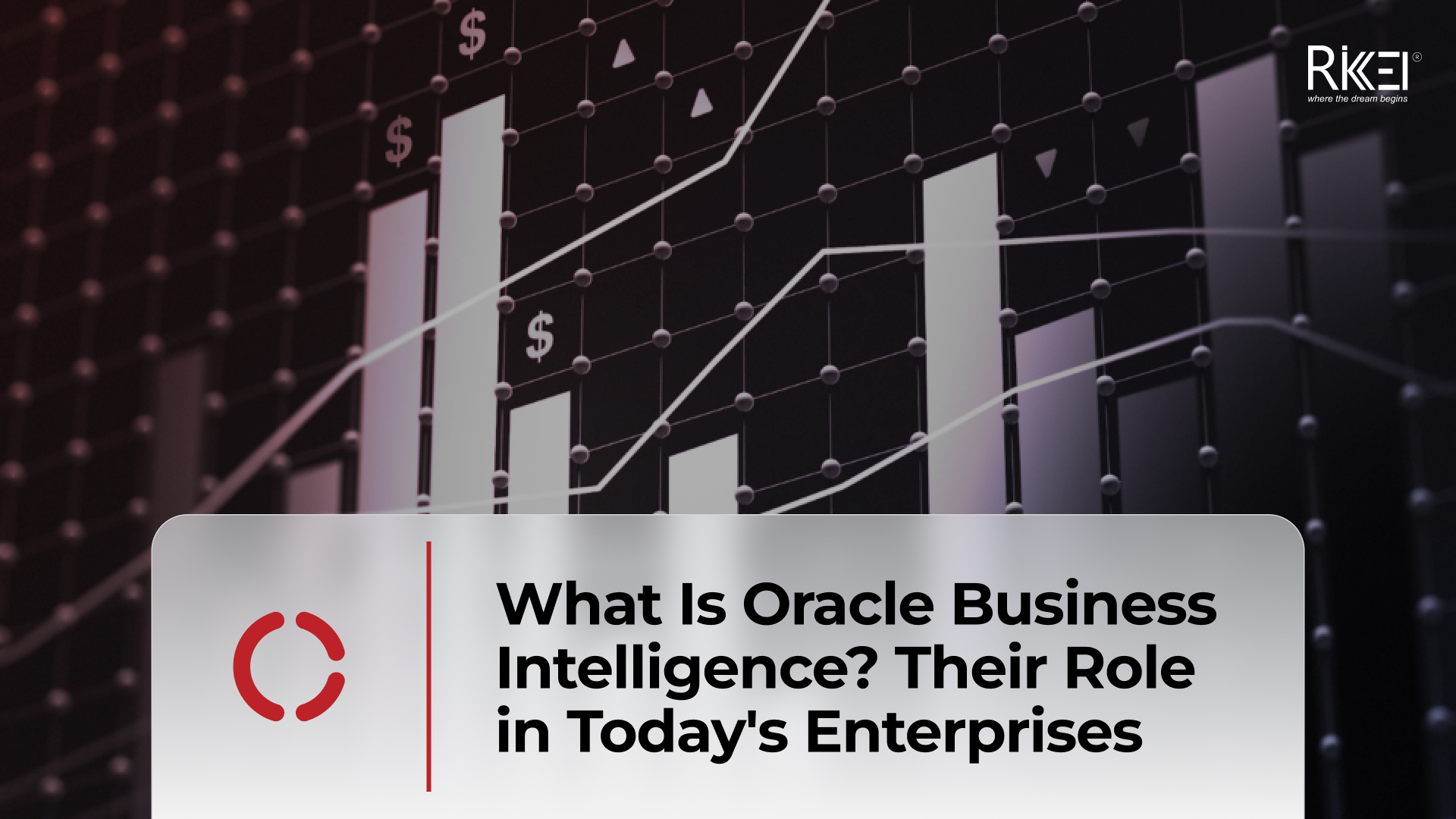
March 14, 2024
What Is Oracle Business Intelligence? Their Role in Today’s Enterprises
Oracle Business Intelligence (BI) refers to a suite of tools, technologies, and applications designed to help organizations collect, analyze and present business data. The primary goal of Oracle BI is to provide actionable insights to support decision-making within an organization. Oracle BI encompasses a range of products that enable users to gather, process and visualize […]
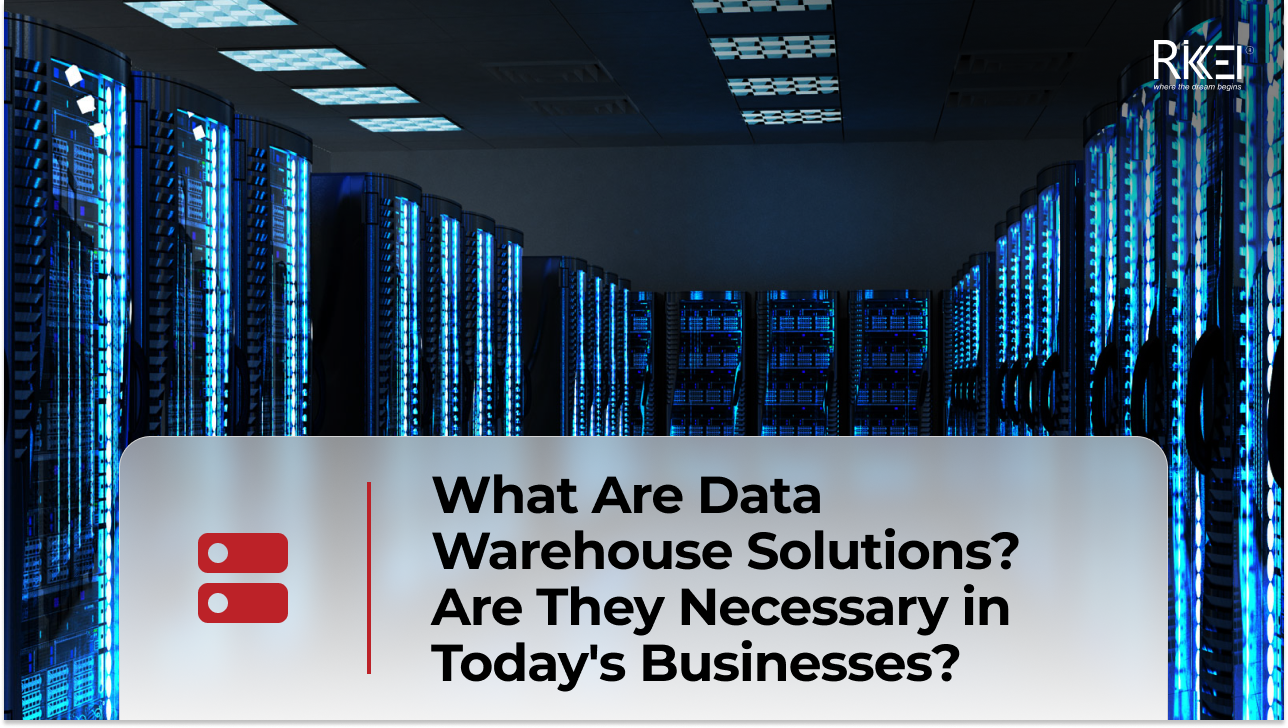
March 7, 2024
What Are Data Warehouse Solutions? Are They Necessary in Today’s Businesses?
Data warehouse solutions are specialized systems designed to efficiently store, retrieve, and analyze large volumes of structured and sometimes unstructured data. The primary goal of a data warehouse is to support business intelligence (BI) activities, such as reporting, querying, and data analysis, by providing a centralized and optimized repository of data. Understanding Data Warehouse Solutions […]