How Real-Time Data Analysis Empowers Your Business
Contents
In today’s fast-paced business landscape, the ability to quickly make data-driven decisions has become a key differentiator for success. Real-time data analysis, the process of analyzing data as soon as it’s generated, has emerged as a powerful tool to empower business across industries. By leveraging real-time data analysis, organizations can gain timely and actionable insights, respond swiftly to changing conditions, and make informed decisions that drive growth and competitive advantage. In this article, we will explore the concept of real-time data analysis, the benefits it brings, and the key steps involved in implementing a real-time data analysis strategy.
What is Real-Time Data Analysis?
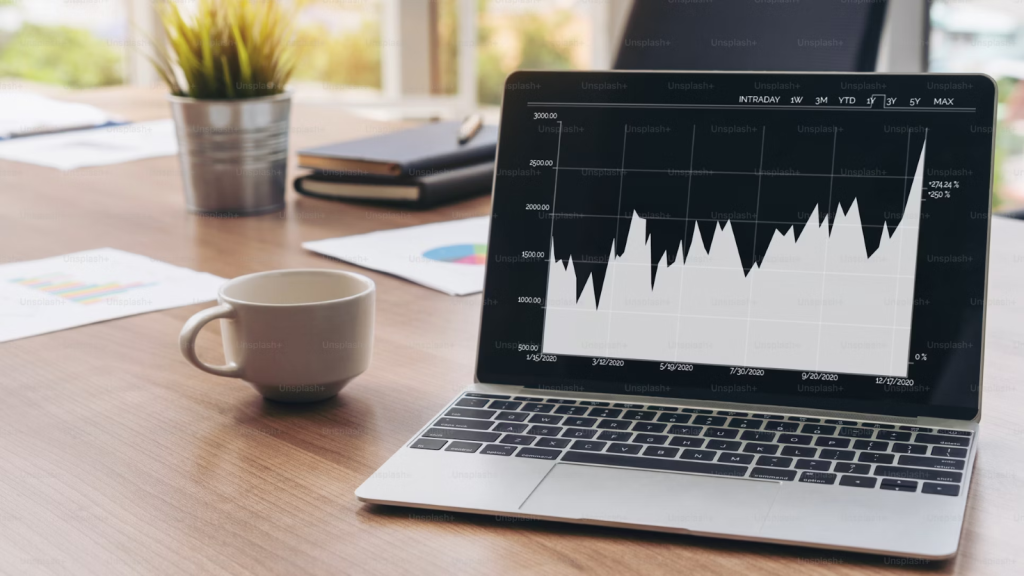
Real-time data refers to information that is collected, processed, and made available for analysis instantaneously or with minimal delay. It represents the most up-to-date and current state of the data. Think of it as a streaming of data flow in real time, where each data point is accessible and can be analyzed immediately.
In the context of real-time data analysis, the data is continuously monitored and analyzed as it arrives, enabling organizations and individuals to gain insights and take prompt actions by. This dynamic and responsive approach is particularly valuable in applications where timely decision-making is crucial, such as financial trading, emergency response systems, and real-time monitoring of critical infrastructure. Real-time data analysis is a powerful tool for business intelligence (BI), like a financial dashboard providing real-time insights. For example, real-time analytics can track revenue trends for companies you’ve invested in and detect any sudden spikes or dips. This allows you to react swiftly, potentially adjusting your investment strategy based on the latest information.
Real-time vs Near-real time analysis
While real-time data analysis provides immediate insights with undeniable advantages, it’s essential to understand how it compares to near-real time technologies. Can’t they be used interchangeably? Let’s explore the key distinctions between these two processing methods and the specific technologies they power.
- Real-time technologies aim to process and analyze data immediately as it is generated, typically within milliseconds or seconds. This allows for immediate insights and actions based on up-to-the-moment data.
- Near-real time analysis refers to processing and analyzing data with a slight delay, usually a matter of seconds, minutes, or hours. It offers a slightly slower but still timely analysis of data.
So, how do these two compare? The key difference lies in the time lag involved. Real-time data analysis operates with minimal latency, enabling instantaneous access and analysis of data as it arrives. On the other hand, near-real time analysis introduces a slight delay to allow for data collection, aggregation, and processing before analysis takes place.
Real-time data analysis is designed to provide the most current and actionable insights, often in time-critical scenarios, benefiting businesses that need to act on data right away. For instance, systems like self-driving cars and air traffic control rely on machine vision memory to analyze their surroundings in real-time, allowing them to make quick decisions based on the visual information they capture. Near-real time analysis, while not instantaneous, still delivers valuable insights with a slight time lag, making it suitable for applications where immediate action is not required but timely decision-making is still important. Inventory management in a retail store is a good example – understanding sales trends with a slight delay can still inform decisions about restocking.
Even a small-time lag can make a difference depending on the application. However, both real-time and near-real time analysis offer significant advantages over traditional methods that rely on historical data analysis. Organizations need to evaluate their needs, the criticality of time-sensitive insights and the feasibility of implementing both approaches to determine the best for their analysis need.
Benefits of Real-Time Data Analysis for Businesses
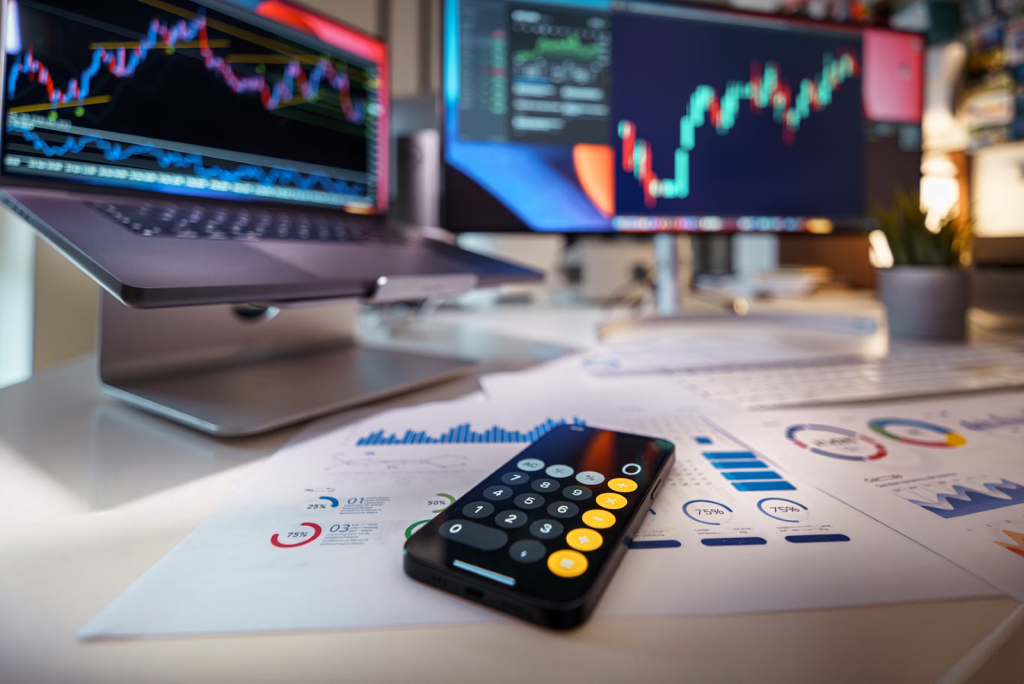
Real-time data analysis offers several benefits to businesses, empowering them to make informed decisions, respond promptly to changing conditions, and gain a competitive edge. Here are some key benefits of real-time data analysis:
- Improved decision-making speed: With real-time insights, businesses can make data-driven decisions faster and more confidently, empowering them to move forward on their decisions, regardless of their scale. They can analyze customer behavior, market trends, and operational metrics as they happen, allowing for agile adjustments to strategies and tactics.
- Proactive problem solving: Issues can be identified and addressed before they escalate into major problems. Predictive maintenance, for example, can be implemented based on real-time sensor data from equipment, preventing costly downtime.
- Fraud Detection: Real-time data analysis is valuable for risk management and fraud detection. By continuously monitoring data streams, businesses can detect suspicious activities, potential security breaches, and fraudulent transactions in real-time, enabling proactive measures to mitigate risks and protect their assets.
- Enhanced user experience and satisfaction: Real-time data analysis helps businesses gain insights into customer behavior, preferences, and sentiment as it happens. This enables personalized marketing campaigns, real-time customer support, and the ability to respond promptly to customer feedback and concerns, ultimately enhancing the overall customer experience.
- Increased Efficiency and Productivity: Generating reports and analyzing data manually took a lot of time and effort. By identifying bottlenecks and inefficiencies in real-time, businesses can optimize operations, streamline processes, automate report generation and improve resource allocation. Employees now can dedicate their time to higher-level tasks. This translates to cost savings and a more productive workforce.
- Accurate Revenue Tracking and Financial Insights: Real-time data analysis is instrumental in tracking revenue, gaining accurate financial insights and ensuring overall financial health of businesses. This allows for immediate visibility into sales performance, revenue growth, and potential revenue leakage. Real-time data analysis also enables businesses to identify cross-selling or upselling opportunities in real-time, optimizing revenue generation and predicting future income.
In short, real-time data analysis empowers businesses with immediate insights, agility, and the ability to make data-driven decisions in rapidly changing environments. By leveraging real-time data, organizations can optimize operations, enhance customer experiences, mitigate risks, and gain a competitive advantage in today’s fast-paced business landscape.
Examples of Real-Time Data Analysis
Real-time data analysis is revolutionizing how businesses operate in a variety of industries. Let’s check out some examples of how entrepreneurs in different industries leverage real-time data analysis for success:
Retail
- Optimizing Inventory Management: Major retail chains use real-time sales data to track inventory levels across stores. This allows them to identify popular items and restock quickly, preventing stockouts and lost sales. Additionally, they can analyze trends to predict future demand and optimize purchasing decisions.
- Personalized Product Recommendations: E-commerce platforms leverage real-time customer behavior data (browsing history, past purchases) to recommend products in real-time. This personalized approach increases customer engagement and conversion rates.
- Dynamic Pricing: Some retailers use real-time data on competitor pricing, customer demand, and market trends to adjust prices dynamically. This allows them to stay competitive and maximize profit margins.
For example, Walmart, the world’s retail giant, recognized the limitations of analyzing past sales data. They built a massive private cloud to handle a staggering amount of data – 2.5 petabytes every hour. This real-time data stream allows them to gain insights much faster than traditional methods that rely on weekly or monthly sales reports. In essence, Walmart leverages real-time data analysis to achieve continuous intelligence. This enables them to make decisions and react to situations much more quickly, potentially leading to increased sales and improved customer experiences.
Finance
- Financial Operations: Financial institutions are undergoing a major shift beyond traditional back-office tasks, taking on more strategic role and providing more valuable insights that drive business decisions. Accurate financial statements are crucial for informed decision-making, and real-time analytics empower teams to identify errors and minimize operational risks. Modern financial software plays a key role in this transformation. By automating tasks like account reconciliation, ensuring secure data storage, and transforming raw data into real-time insights, these tools empower teams to stay accurate, agile, and ahead of the curve.
- High-Frequency Trading: Investment firms leverage real-time market data to make rapid buy and sell decisions in the stock market in milliseconds. Real-time analytics equip financial institutions with a comprehensive view of the market. By instantly analyzing data from financial databases, news feeds, social media and even weather reports, they can identify trends and make informed trading decisions based on the complete picture.
- Personalized Investment Advice: Financial advisors can use real-time data on a client’s portfolio performance, market trends, and risk tolerance to provide personalized investment recommendations and adjust as needed.
For instance, Capital One, a diversified financial services company based in the US, has leveraged real-time data analysis to enable it to make data-driven decisions in areas such as fraud protection, customer marketing, product offerings and risk management. The adoption of Snowflake’s Data Cloud has enabled Capital One to achieve high performance and elasticity in their analytics operations. They can now accommodate thousands of analysts running millions of queries simultaneously without compromising performance.
Manufacturing
- Predictive Maintenance: Factories use real-time sensor data from machines to predict equipment failures before they occur. This proactive approach minimizes downtime, reduces maintenance costs, and ensures smooth production processes.
- Real-Time Quality Control: Manufacturers can integrate real-time data from sensors on production lines to monitor product quality throughout the manufacturing process. This allows them to identify and address defects immediately, improving product quality and reducing waste.
- Supply Chain Optimization: Real-time data on inventory levels, production status, and transportation can be used to optimize supply chains. This allows manufacturers to respond to disruptions efficiently and ensure on-time delivery of products.
For example, General Electric utilizes real-time data analysis to optimize and streamline their manufacturing operations. Sensors embedded in their equipment constantly feed data on production, equipment performance, and supply chain dynamics. This real-time analysis allows GE to identify inefficiencies, predict potential equipment issues, and optimize production schedules. By leveraging these insights, GE can minimize downtime, improve overall efficiency, and make data-driven decisions.
Whether it’s personalizing customer experiences, mitigating risks, optimizing operations, or improving decision-making, businesses are harnessing the power of real-time data analysis to gain a competitive edge and achieve their goals. As real-time data analysis evolves, we can expect to witness its possibilities to constantly expand and gain a significant competitive edge.
Getting Started with Real-Time Data Analysis
You don’t necessarily need cutting-edge technology to get started with real-time analytics, a standard computer with internet access can suffice. You can also leverage tools like R or Python on your local network to analyze incoming data streams in real-time. This analysis can be done by a data analyst at their workstation, or even automated by a machine learning model running on a server, either in your office or remotely.
Here’s a breakdown of how real-time data analysis works to get you started:
- Identify Your Data Sources: The first step is understanding what data you have and where it comes from. This could include customer transactions, website traffic logs, social media feeds, sensor data from IoT devices, or financial market data feeds. It is also important to understand the structure, format and frequency of data generated by each source to evaluate the quality and reliability of the data sources.
- Choose the Right Tools & Technologies: There are various tools and technologies available for real-time data analysis. Some factors to consider when choosing include the volume and velocity of your data, your budget, and your technical expertise. Popular options include streaming platforms like Apache Kafka, real-time analytics tools like Apache Spark, and data visualization dashboards like Power BI or Tableau. Moreover, considering cloud-based solutions that provide scalability, reliability and real-time processing capabilities, such as Google Cloud, Azure…
- Develop a Data Analysis Strategy: Don’t jump straight into analysis! Define clear goals for what you want to achieve with real-time data. What questions are you trying to answer? What insights are you hoping to gain? This step may include determining the analytics techniques and algorithms (machine learning models, detection algorithms or statical methods…), designing data processing pipelines to handle real-time data ingestion, transformation and analysis, and implementing real-time monitoring to track data quality, system performance and anomalies.
- Data Collecting and Processing: It’s crucial to set up data collection mechanisms to gather real-time data from the identified sources. This may involve integrating with APIs, setting up data connectors, or implementing data ingestion pipelines. Before processing, developing a data processing workflow to handle real-time data streams and perform data transformations. It’s important to ensure data security and privacy measures are in place to protect sensitive information during data collection and processing.
- Data Visualization: After processing, the data is then subjected to various analyses, visualization tools can be applied to represent your real-time insights in a clear and actionable way. Real-time dashboards and interactive visualizations allow you to monitor key metrics, identify trends, and make data-driven decisions quickly. This also enables stakeholders to understand and act upon real-time insights effectively.
- Iterate and Refine: Real-time data analysis is not a one-time process, it is an ongoing process. As you gather more data and gain insights, you may need to refine your strategy, update your analytic model, adjust your tools, and adapt your visualizations to any changes in data patterns and business requirement over time. The key is to be flexible and continuously improve your real-time data analysis practices.
To put it briefly, real-time data analysis involves a combination of technologies and procedures aimed at rapidly transforming raw data into valuable insights. Throughout the entire process, starting from identifying data sources to the presentation of actionable outcomes, the objective is to equip businesses with the ability to make prompt and well-informed decisions admist an ever-evolving landscape.
Conclusion
Real-time data analysis has become a game-changer for businesses, enabling them to thrive in the era of big data and digital transformation. By harnessing the power of real-time insights, organizations can make informed decisions, optimize operations, enhance customer experiences, and gain a competitive edge in their respective industries. The ability to monitor, analyze, and act upon data in real-time has revolutionized the way businesses operate, enabling them to adapt swiftly to changing market dynamics, identify emerging opportunities, and mitigate risks proactively.
More From Blog
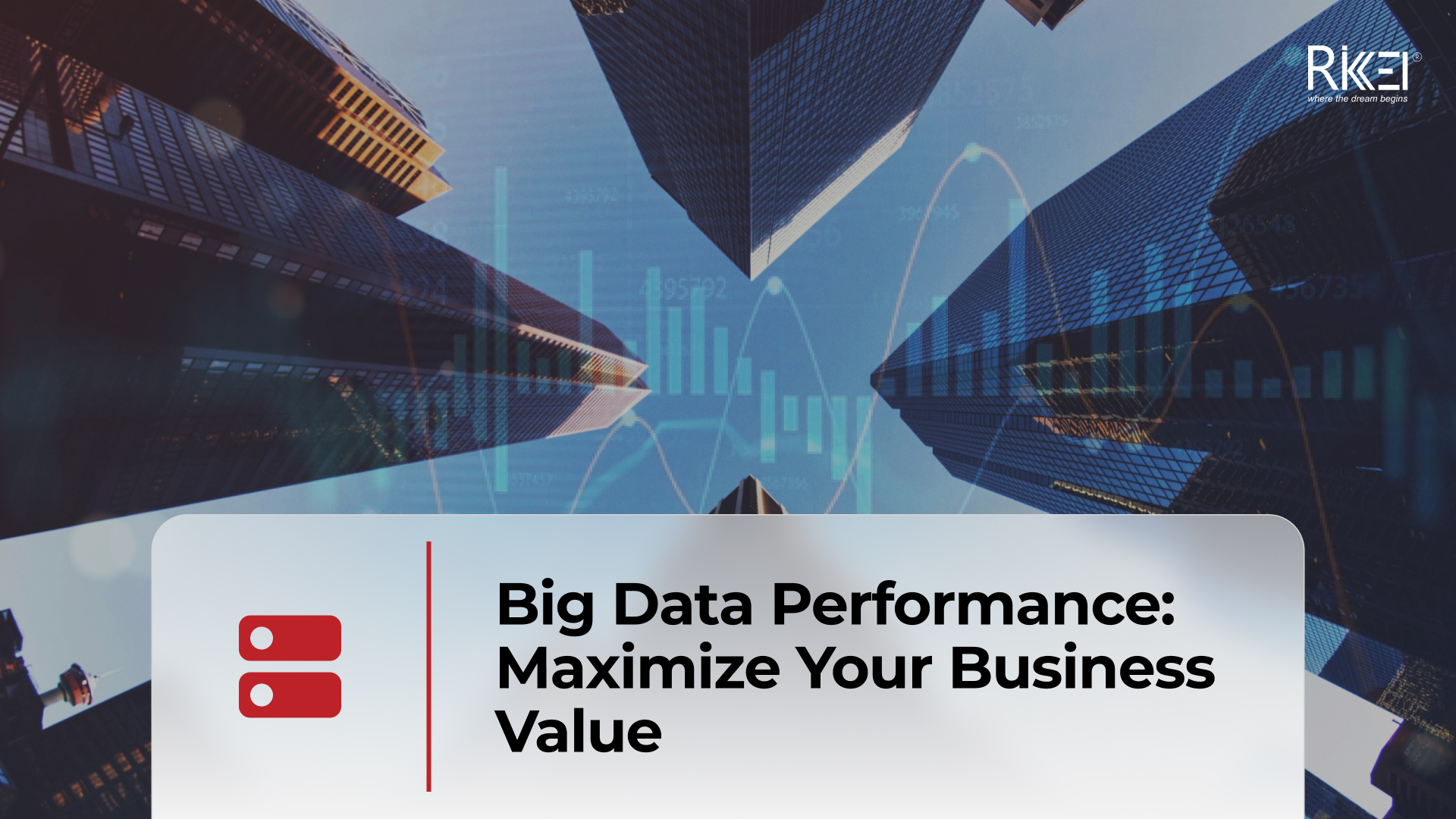
April 4, 2024
Big Data Performance: Maximize Your Business Value
In today’s data-driven world, organizations are constantly generating and collecting immense amounts of data to understand their customers more deeply. This data, often referred to as “big data,” holds immense potential for organizations to seek opportunities and overcome challenges. But accessing and analyzing big data isn’t enough to have proper strategies; organizations must pay attention to […]
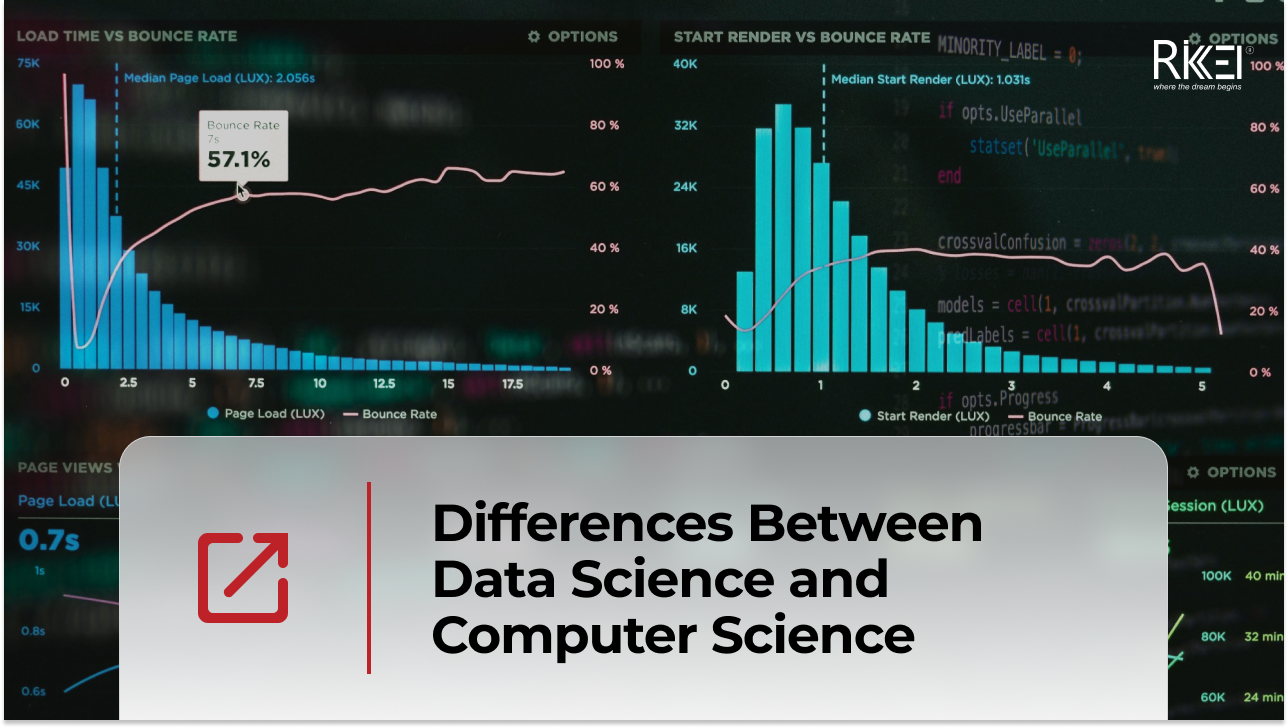
April 4, 2024
Differences Between Data Science and Computer Science
Data Science and Computer Science are distinct fields overlapping in certain areas but have different focuses and objectives. The article below will help you clearly understand the differences and the close connection between the two fields. What is Data Science? Data Science is an interdisciplinary field that combines scientific methods, processes, algorithms, and systems to […]
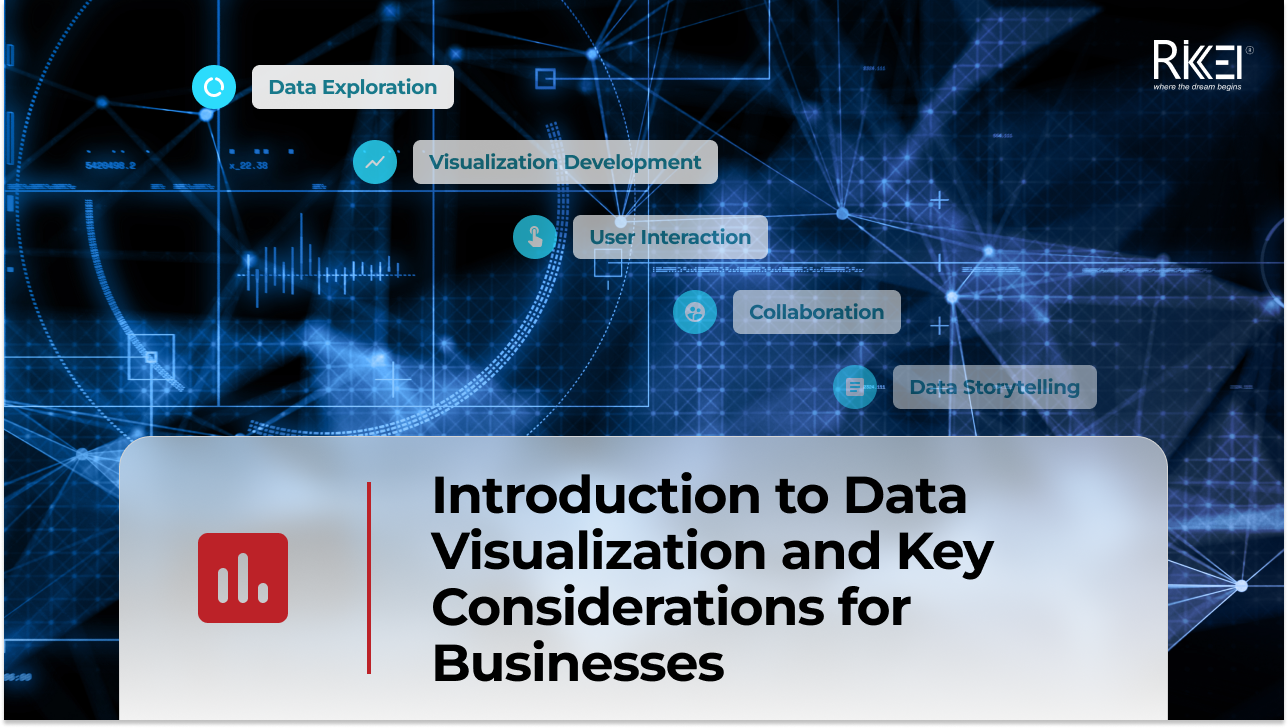
March 28, 2024
Introduction to Data Visualization and Key Considerations for Businesses
In your opinion, what is data visualization? Your main goal is to communicate your recommendations engagingly and effectively, right? To achieve this, let’s immediately explore a method that can represent information with images. What is Data Visualization? Define data visualization and their roles in organizations First, you need to find the answer to the question: […]
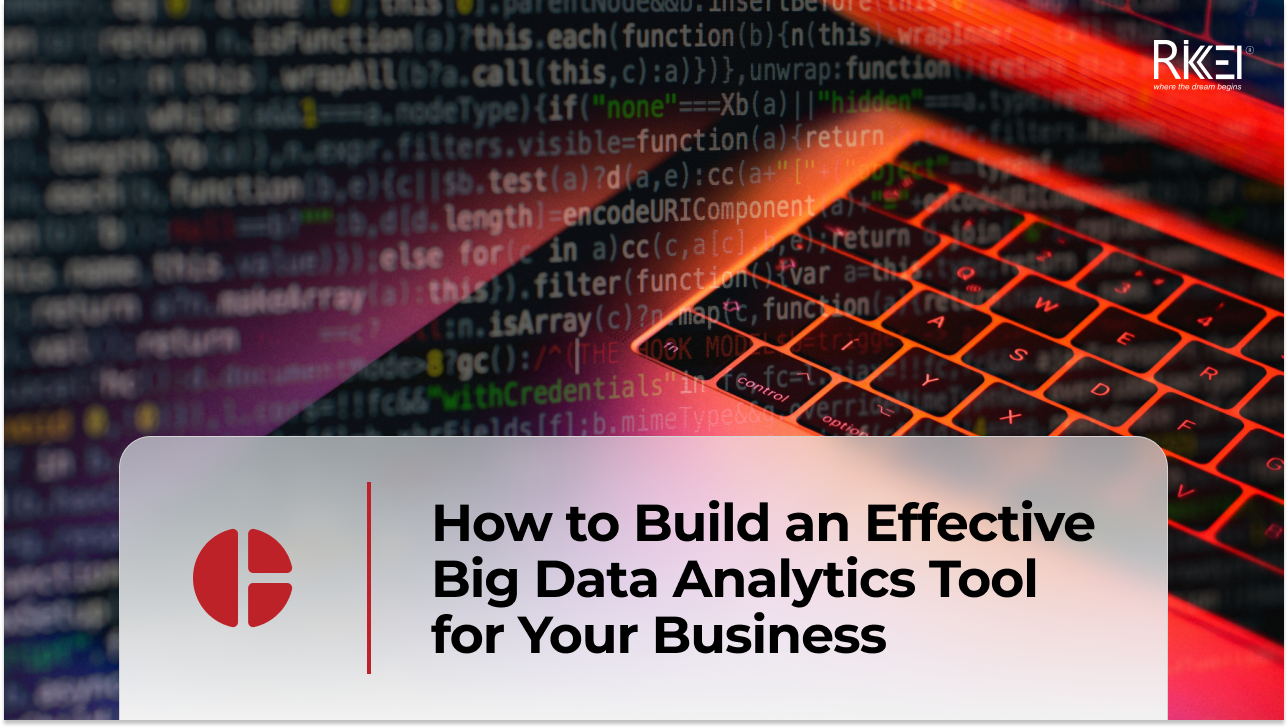
March 21, 2024
How to Build an Effective Big Data Analytics Tool for Your Business
Building an analytics tool for a business brings several significant benefits, especially in today’s business environment where data is becoming larger and more complex. So how to build an effective analysis tool for businesses, follow the article below! Assessing Business Needs Assessing business needs involves understanding the requirements, goals, and challenges of a business or […]
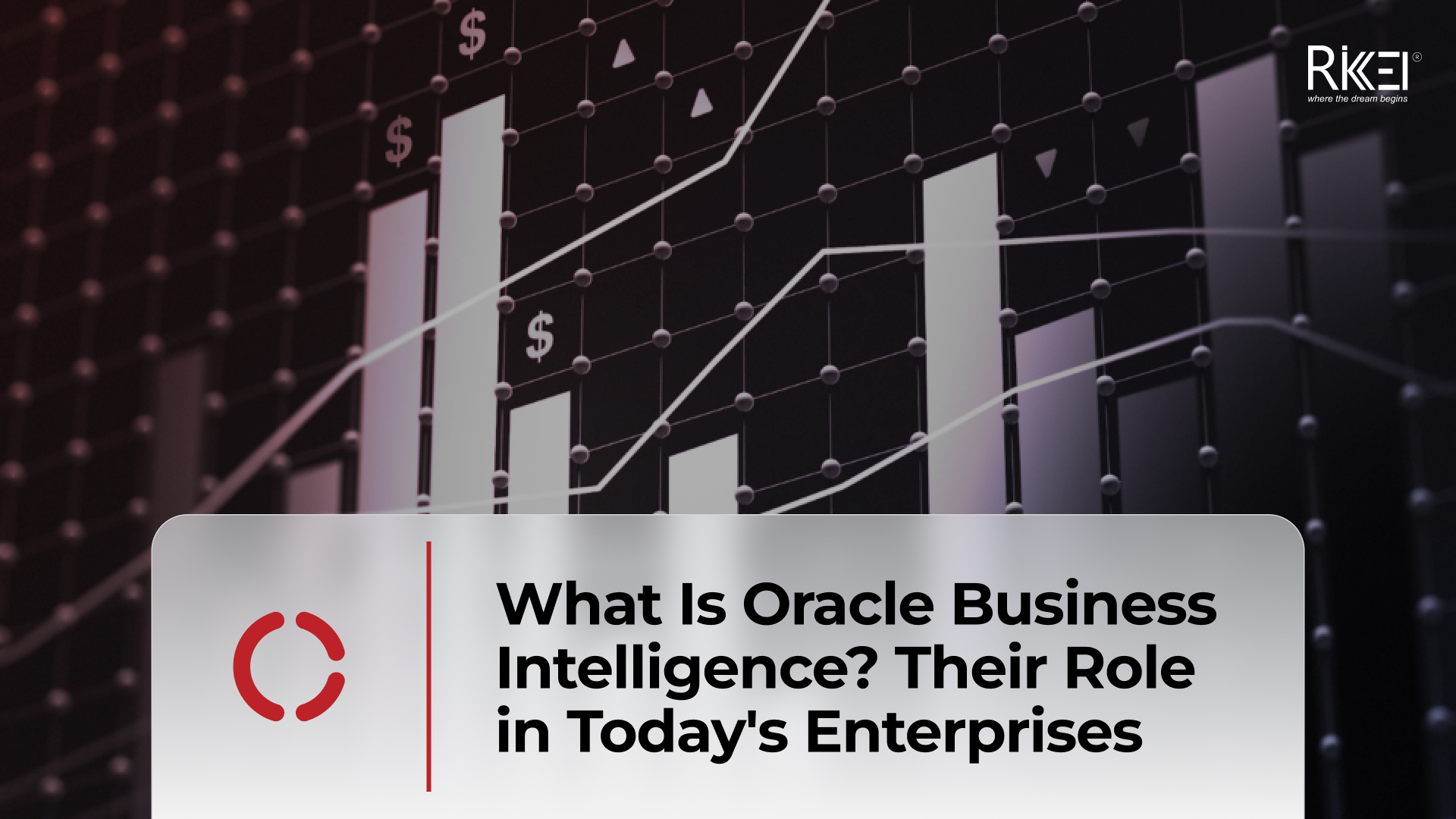
March 14, 2024
What Is Oracle Business Intelligence? Their Role in Today’s Enterprises
Oracle Business Intelligence (BI) refers to a suite of tools, technologies, and applications designed to help organizations collect, analyze and present business data. The primary goal of Oracle BI is to provide actionable insights to support decision-making within an organization. Oracle BI encompasses a range of products that enable users to gather, process and visualize […]
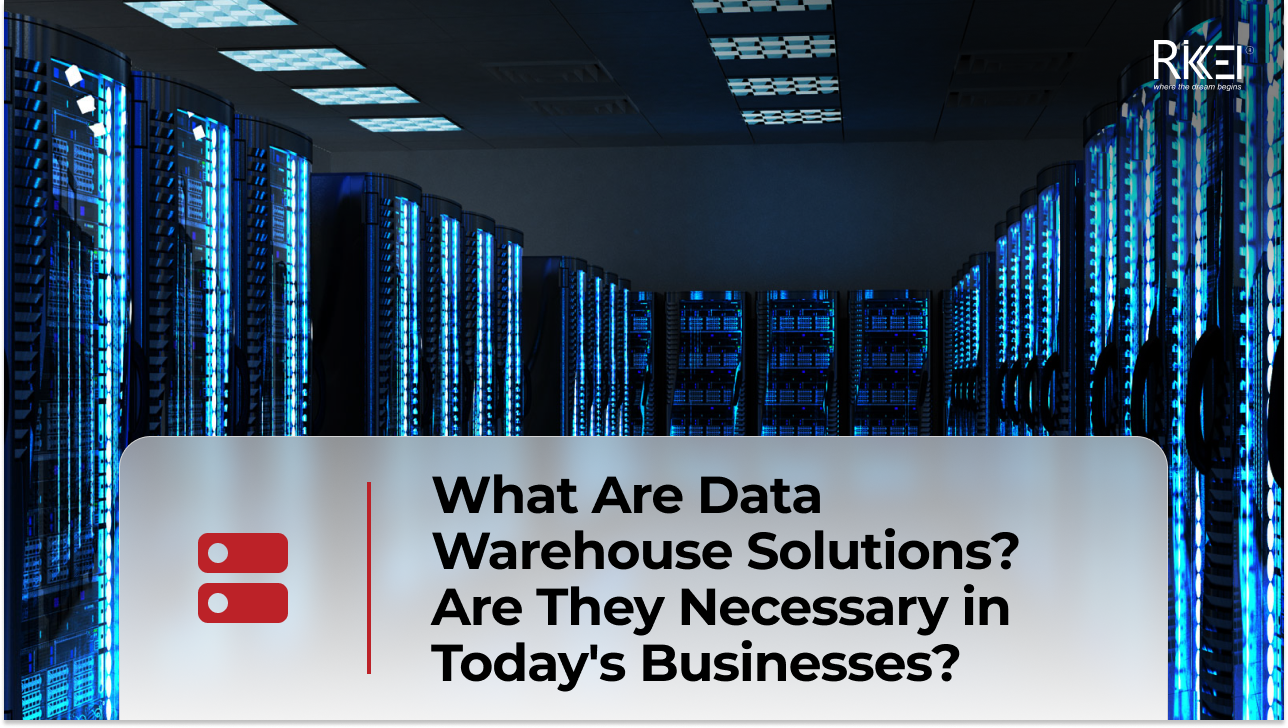
March 7, 2024
What Are Data Warehouse Solutions? Are They Necessary in Today’s Businesses?
Data warehouse solutions are specialized systems designed to efficiently store, retrieve, and analyze large volumes of structured and sometimes unstructured data. The primary goal of a data warehouse is to support business intelligence (BI) activities, such as reporting, querying, and data analysis, by providing a centralized and optimized repository of data. Understanding Data Warehouse Solutions […]