Data-Driven Business Strategy: Using Analytics to Drive Growth
Contents
Organizations today have more data at their fingertips than ever before. But many struggle to actually make full use of it to inform business strategies and decisions. Adopting a data-driven approach is key to remaining competitive and unlocking growth in the modern market.
For companies new to using analytics, the idea of becoming a data-driven business can seem daunting. This article explains what it means to employ a data-driven business strategy along with real-world examples of success.
What is Data-Driven Business Strategy?
In modern business, a data-driven strategy represents a shift from intuition-based decisions or isolated observations, to an approach where every strategic direction is anchored on data-derived insights.
This transformation into a data-centric mindset is not merely about the accumulation of massive data sets. It also involves the meticulous interpretation of data to drive operational efficiency.
The genesis of data-driven business strategies is linked to the digital age. This is where the ubiquity of data collection and the advancements in analytical tools have provided unprecedented opportunities for businesses to leverage data.
It’s an acknowledgment that in the vast sea of information, there are patterns and revelations that, when properly analyzed, can lead to better decisions.
Data can accommodate the planning process every step of the way. Image: Freepik
Benefits of Data-Driven Business Strategy
Implementing a data-driven approach comes with numerous advantages that directly impact bottom lines and competitive positioning.
Measurable Business Goals
Traditionally, objectives may have been set based on historical performances or industry benchmarks. This often leads to aspirational targets without a clear path to achievement.
Data-driven strategies, however, utilize key performance indicators (KPIs) derived from data analytics. They make sure that goals are not only grounded in reality but are also tightly aligned with the company’s overarching strategic vision.
With the rich insights garnered from data, organizations can dissect and understand the nuances of their operational efficiency, customer behavior, and market trends. This granular understanding enables the crafting of specific, measurable, achievable, relevant, and time-bound (SMART) goals.
In turn, the progress towards these goals can be tracked with real-time data, providing an ongoing assessment that is far more dynamic and actionable than annual or quarterly reviews common in traditional strategies.
More Accurate Analyses & Predictions
In a data-driven analytics framework, the accuracy of analyses and the power of predictive analytics surpass what was achievable in traditional approaches.
Historically, companies often relied on aggregated data and forecasting methods that could not account for complex market dynamics or customer behaviors. Data-driven analysis slices through these limitations by leveraging advanced algorithms and machine learning to identify patterns that would be invisible to the human eye.
Predictive analytics has evolved from a novel capability to a critical asset. It enables businesses to anticipate market changes, consumer needs, and potential risks with a higher degree of confidence.
This view is grounded in historical data trends and predictive modeling, which can account for a multitude of variables and ‘what-if’ scenarios. Companies are thus equipped to make informed decisions about product launches, market expansions, and other strategic moves with a predictive foresight that is backed by data rather than merely reacting to market forces or competitors’ actions.
Faster Actions
Speed is a crucial competitive edge in today’s fast-paced market, and a data-driven strategy significantly reduces the time from insight to action.
Traditional decision-making processes often involve lengthy deliberations, extensive market research, and a series of approvals that can delay response times. In contrast, a data-driven approach provides real-time insights that enable quicker decisions.
The ability to act faster is not just about speed for its own sake but also about being responsive to customer demands and market shifts.
Data-driven businesses can adjust their strategies in real time, ensuring that their actions are relevant and timely. This responsiveness can be the difference between capitalizing on a market opportunity or falling behind more agile competitors.
Data can provide a clearer path toward business goals. Image: Freepik
Increased Efficiency & Agility
With accurate data, companies can streamline operations, eliminate wasteful practices, and optimize resource allocation. This level of efficiency is not typically achievable with traditional approaches, which may rely on generalized assumptions and outdated information.
On top of that, it also enhances agility when a business can pivot quickly based on data insights. The rigidity of long-term plans could make it difficult to adapt to change. Data-driven organizations, however, benefit from a more flexible approach that allows them to shift gears and change direction with minimal disruption.
Explores New Opportunities
Data-driven strategies are adept at illuminating paths to new opportunities that might otherwise remain hidden.
They can analyze trends, customer behaviors, and market conditions with sophisticated data tools so businesses can identify unmet needs or emerging niches. This process often reveals innovative product ideas, potential areas for expansion, or underserved customer segments. As a result, companies invest in new ventures with a higher degree of confidence.
Improved Employee Engagement and Loyalty
Data empowers employees by providing clear metrics for success and a transparent way to track progress and contributions. This kind of environment not only fosters a sense of ownership and pride in one’s work but can also deepen loyalty to the company.
Employees are more likely to engage in problem-solving and innovation when they have access to information that supports their ideas and initiatives. Those who feel their input is valued and see a direct impact on their work are also more likely to remain committed to the organization.
Challenges When Implementing Data-Driven Strategy
Even pioneering organizations at the forefront of data analytics can run into common challenges and pitfalls when working to become data-driven.
Compromised Decision-Making Due to Poor Data Quality
Poor data quality—be it inaccurate, incomplete, or outdated—can lead to misguided insights and erroneous decisions. Organizations often struggle with maintaining data integrity, especially when integrating data from various sources. This can result in a lack of confidence in the data and render it more of a liability than an asset in the decision-making process.
Deficiencies in Infrastructure and Analytical Tools
The backbone of this approach is the infrastructure and tools that collect, store, and analyze data.
However, a common obstacle is that businesses may not have the necessary systems in place, or their existing infrastructure is ill-equipped to handle large volumes of data or complex analytics. Without the proper technological foundation, organizations can find it challenging to tap into the full potential of the data at hand.
Data is useless without adequate tools to collect and draw insights from it. Image: Freepik
Resistance to Change in Organizational Culture
Implementing a data-driven strategy often requires a cultural shift within the organization, which can be met with resistance. Employees accustomed to traditional decision-making processes may be skeptical of relying on data analytics. They might even perceive it as a threat to their expertise or established ways of working.
Influence of Cognitive Biases
Cognitive biases like confirmation bias and groupthink pose a significant hurdle in the path to adopting a data-driven strategy.
The former tendency can lead individuals to favor information that corroborates their preconceptions, while groupthink can result in the entire team making flawed decisions in pursuit of consensus. These biases can skew interpretation and overshadow objective data analysis, affecting the strategic decisions that are intended to be rooted in unbiased data.
Case Studies of Data-Drive Business Strategy
Data-driven business strategy is far more than just a theoretical concept – it has been implemented effectively across industries and around the globe, with many outstanding success stories exemplifying its tangible benefits.
Netflix’s Data-Driven Success with “House of Cards”
The hit show “House of Cards” is one of the biggest examples of data driven business case studies across strategy marketing. In particular, it makes the case for big data as a potent tool for curating and commissioning content that resonates with audiences worldwide.
Armed with an expansive collection of viewing data, Netflix identified a unique opportunity. They observed that their subscribers not only gravitated towards David Fincher’s directorial style, evidenced by the viewership of “The Social Network,” but also had a keen interest in political dramas, as shown by the consistent streaming of the British “House of Cards” series. Also, films featuring Kevin Spacey were reliably popular among the audience.
With these insights, Netflix’s leadership, including Chief Communications Officer Jonathan Friedland, surmised that an American adaptation of “House of Cards” would appeal to a significant portion of their user base.
Upon the series’ release, Netflix also deployed a tailored marketing strategy, where viewers received customized trailers based on their past viewing habits—a move that ensured higher engagement rates.
The show “House of Cards” is a prime example of data-driven film production. Image: YouTube
The result was an unequivocal success. “House of Cards” not only became the most-streamed piece of content in the United States and across 40 other countries, but it also cemented Netflix’s reputation as a disruptive force in the entertainment industry.
Starbucks’ Pumpkin Spice Latte Campaign
The annual release of Starbucks’ Pumpkin Spice Latte heralds the onset of autumn for many consumers. It embodies a seasonal tradition that drives significant foot traffic to stores and is typically marked by a surge in sales and customer engagement.
However, in 2020, the challenge for Starbucks was formidable. Amidst a global pandemic and with the UK entering a second lockdown, the customary in-store bustle and outdoor advertising campaigns were rendered ineffective.
The urgent commercial goal was to recapture pre-COVID sales levels by March 2021, and the impending Pumpkin Spice Latte season was targeted as the springboard for this recovery.
Faced with the locked-down populace, Starbucks, alongside its partners Havas and MiQ, pivoted to a data-centric approach to tempt the “at-home” audience back into stores.
This strategy was centered on an advanced analysis of anonymized mobile data, a first for MiQ, encompassing user behavior from over 20 million UK handsets. They dissected this information to identify new and potential customers based on location, demographic, and app usage patterns.
The outcomes of this innovative data-driven campaign were telling. Beyond achieving a significant uplift in brand metrics and purchase intent, the strategy notably over-delivered on Pumpkin Spice Latte sales targets during the campaign period.
Starbucks has used data extensively to recover from the pandemic. Image: Pexels
Best Practices in Making Data-Driven Business Strategy
By following key best practices, organizations can ensure a successful rollout of data-driven business strategies and steer clear of pitfalls that trip up others:
- Cultivate a Data-Driven Culture: Encourage an environment where decisions are supported by data. This involves training staff to think critically about data and to utilize it when justifying decisions.
- Prioritize Data Across the Organization: Data must be recognized as a critical asset at every level of the organization. Leaders should champion the use of data, making it central to all strategic planning and operational processes.
- Implement a Data Governance Protocol: Data governance is essential for maintaining data integrity. Establish clear policies for data management to ensure accuracy, security, and compliance with regulatory standards.
- Align KPIs With Business Objectives: Clearly define and consistently track KPIs that are aligned with strategic business goals. This regular monitoring serves as a compass for both short-term actions and long-term strategies.
- Stop Chasing the Latest Trends: While new technologies can be valuable, they should be adopted judiciously. Invest in technologies that align with your specific business needs rather than being swayed by fleeting trends.
- Facilitate Cross-Functional Data Flow: Break down silos and encourage cross-departmental collaboration to ensure data is accessible and leveraged across the organization to enhance overall business intelligence.
Conclusion
Data-driven business strategy can provide transformative benefits for organizations of all types and sizes. With analytics and insights drawn from customer, operational, and market data, you can drive innovation, optimize efficiency, and gain competitive advantages.
While transitioning to a data-focused approach requires investment and commitment, the long-term rewards make it a smart strategy for future-proofing your business.
More From Blog
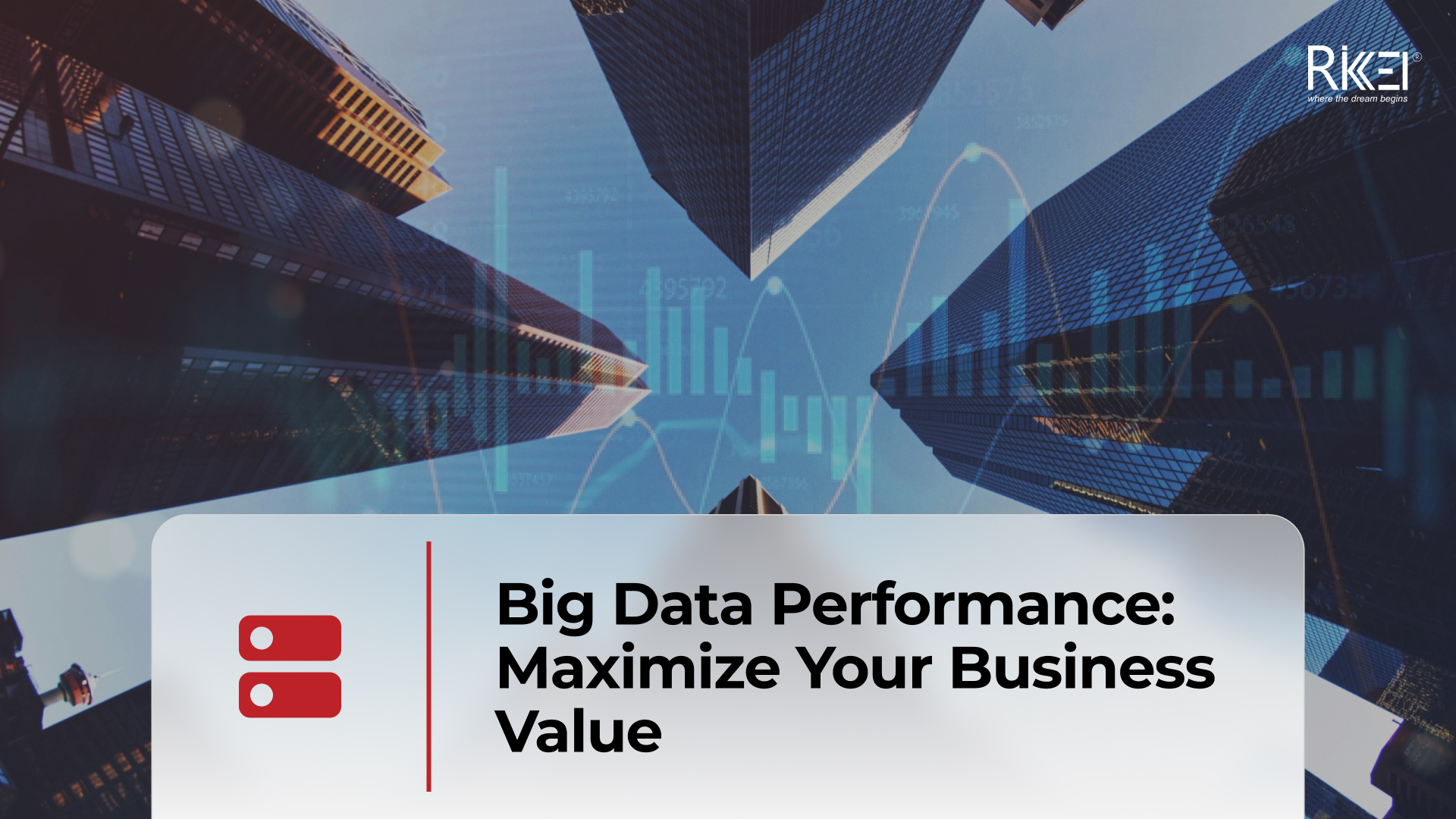
April 4, 2024
Big Data Performance: Maximize Your Business Value
In today’s data-driven world, organizations are constantly generating and collecting immense amounts of data to understand their customers more deeply. This data, often referred to as “big data,” holds immense potential for organizations to seek opportunities and overcome challenges. But accessing and analyzing big data isn’t enough to have proper strategies; organizations must pay attention to […]
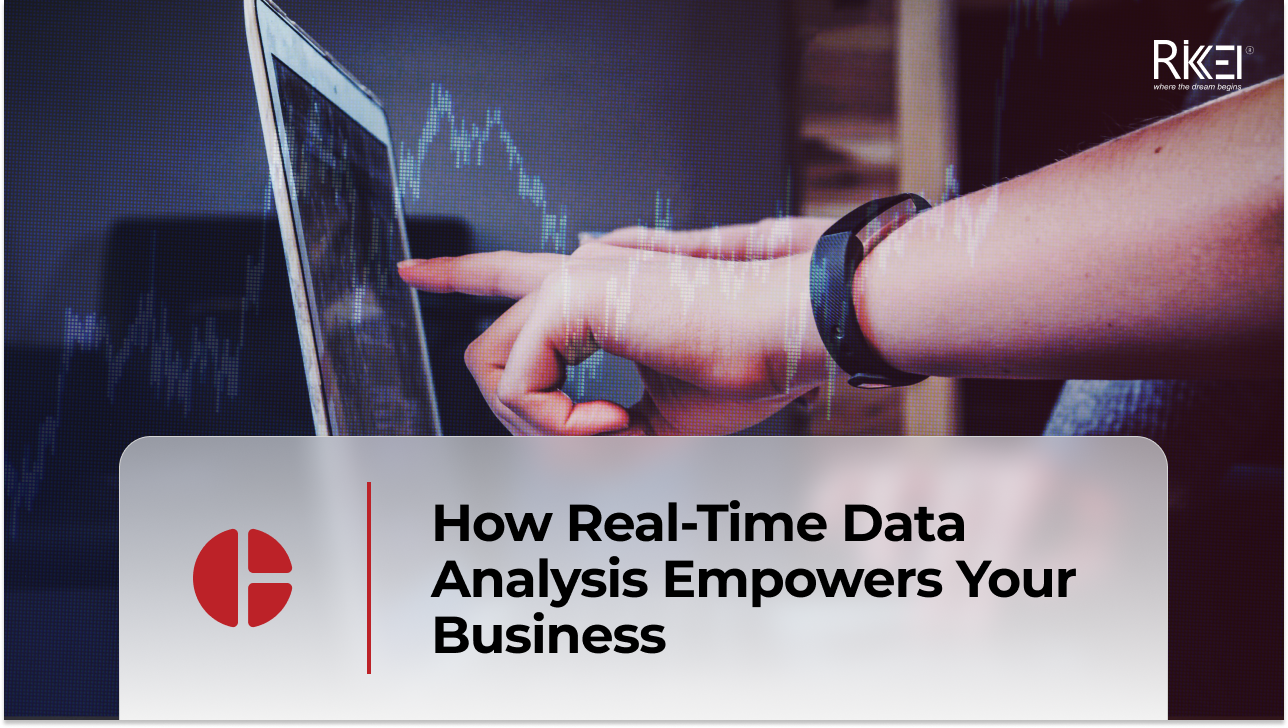
April 4, 2024
How Real-Time Data Analysis Empowers Your Business
In today’s fast-paced business landscape, the ability to quickly make data-driven decisions has become a key differentiator for success. Real-time data analysis, the process of analyzing data as soon as it’s generated, has emerged as a powerful tool to empower business across industries. By leveraging real-time data analysis, organizations can gain timely and actionable insights, […]
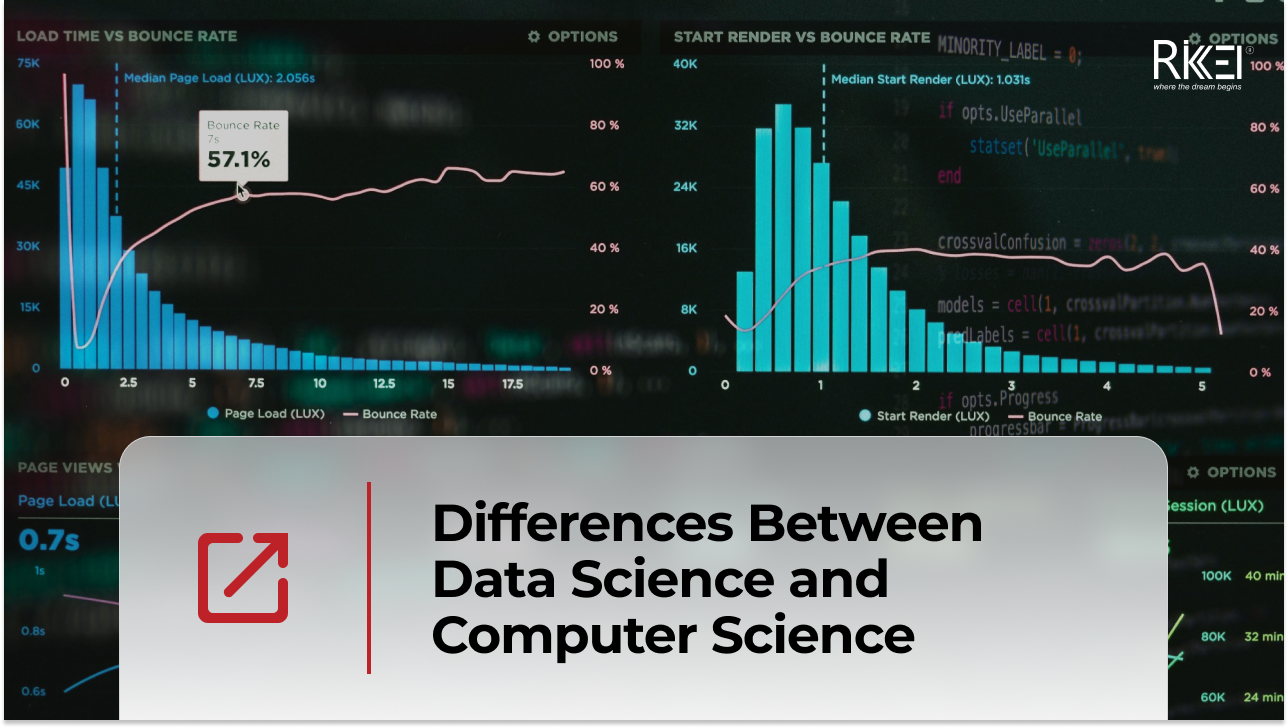
April 4, 2024
Differences Between Data Science and Computer Science
Data Science and Computer Science are distinct fields overlapping in certain areas but have different focuses and objectives. The article below will help you clearly understand the differences and the close connection between the two fields. What is Data Science? Data Science is an interdisciplinary field that combines scientific methods, processes, algorithms, and systems to […]
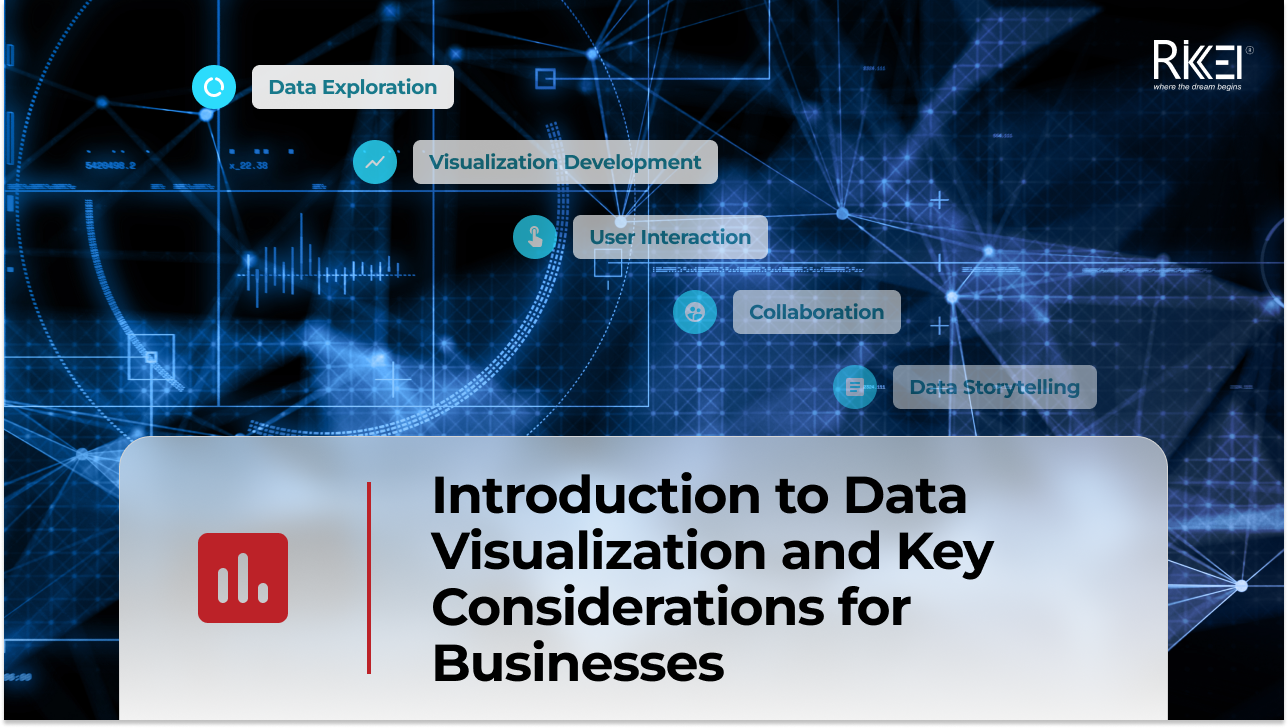
March 28, 2024
Introduction to Data Visualization and Key Considerations for Businesses
In your opinion, what is data visualization? Your main goal is to communicate your recommendations engagingly and effectively, right? To achieve this, let’s immediately explore a method that can represent information with images. What is Data Visualization? Define data visualization and their roles in organizations First, you need to find the answer to the question: […]
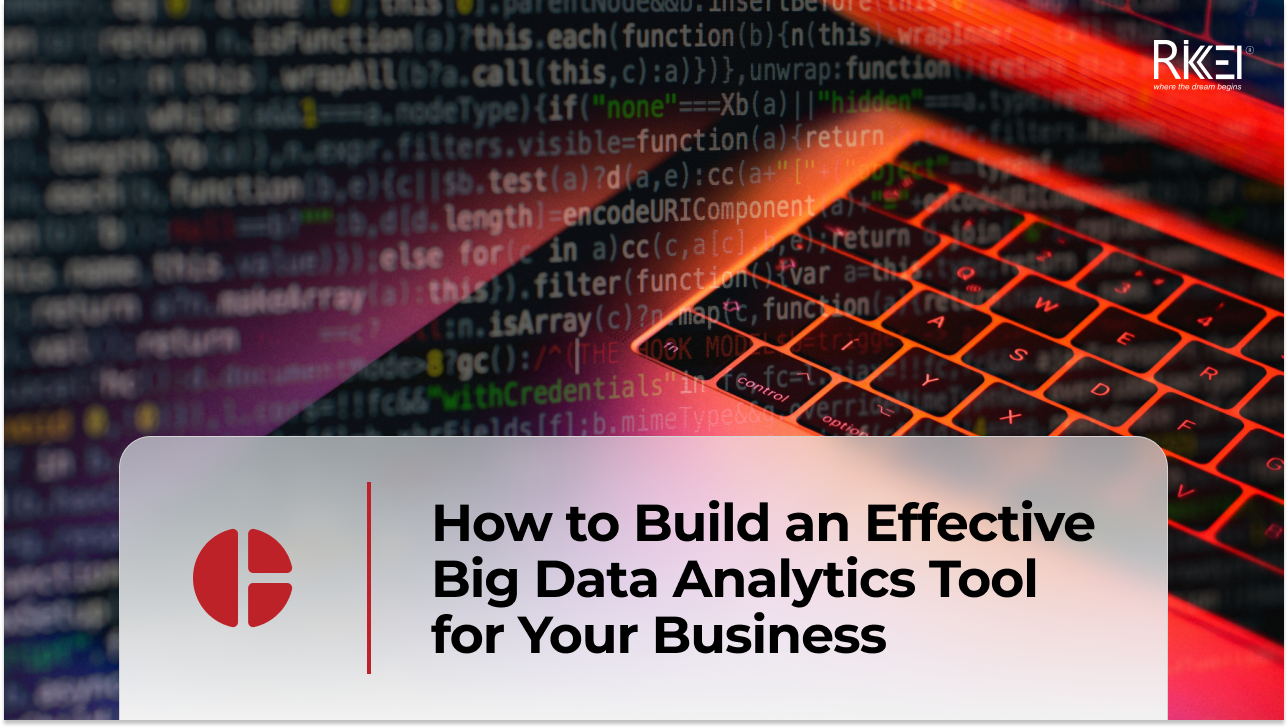
March 21, 2024
How to Build an Effective Big Data Analytics Tool for Your Business
Building an analytics tool for a business brings several significant benefits, especially in today’s business environment where data is becoming larger and more complex. So how to build an effective analysis tool for businesses, follow the article below! Assessing Business Needs Assessing business needs involves understanding the requirements, goals, and challenges of a business or […]
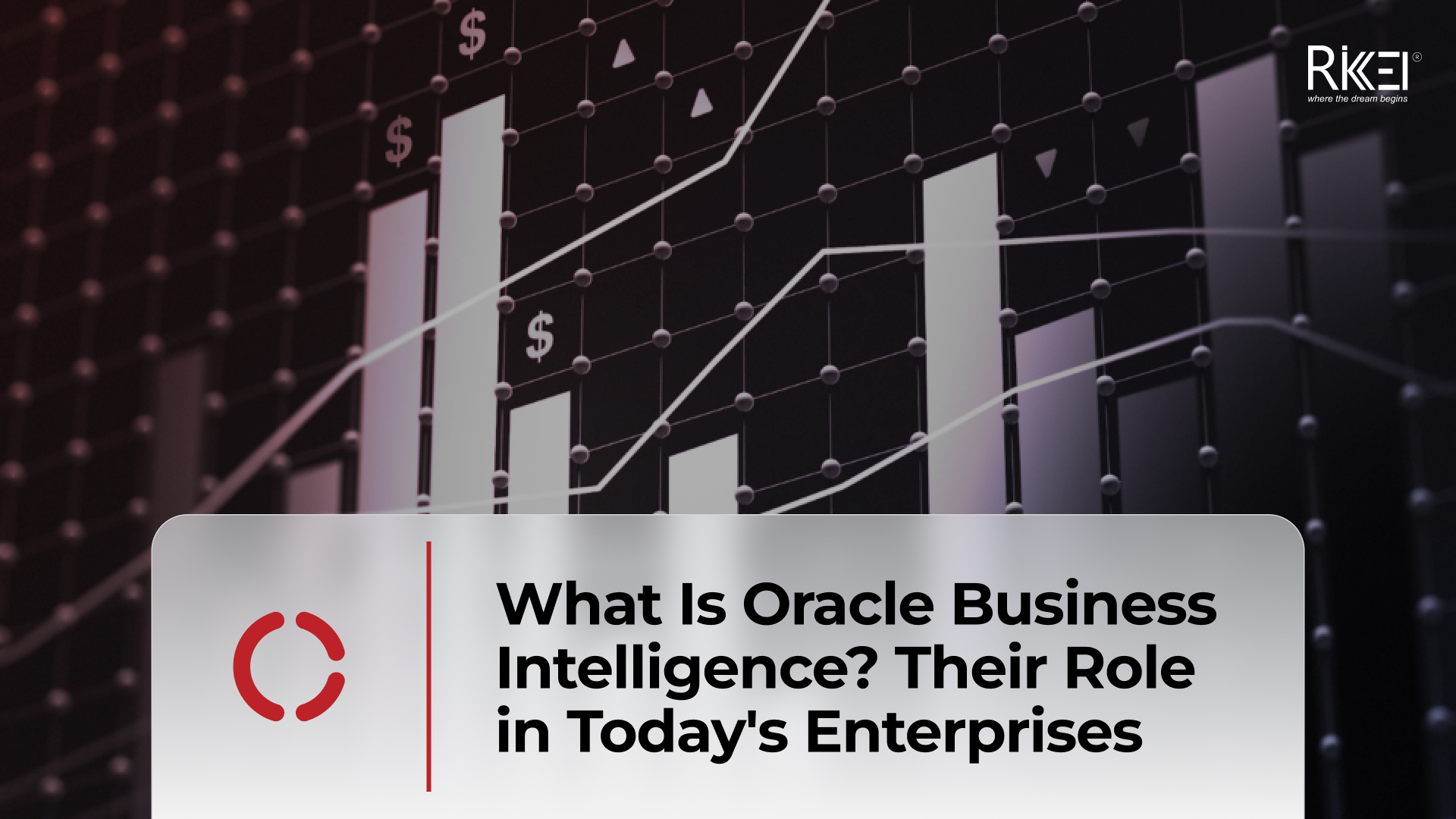
March 14, 2024
What Is Oracle Business Intelligence? Their Role in Today’s Enterprises
Oracle Business Intelligence (BI) refers to a suite of tools, technologies, and applications designed to help organizations collect, analyze and present business data. The primary goal of Oracle BI is to provide actionable insights to support decision-making within an organization. Oracle BI encompasses a range of products that enable users to gather, process and visualize […]