5 Types of Data Analytics Framework
Contents
In today’s digital age, businesses and organizations across industries are generating massive volumes of data. This data contains valuable insights and patterns that, when properly analyzed, can help drive strategic decision-making, optimize operations, and uncover new opportunities. However, the sheer volume and complexity of data make it challenging to extract meaningful information without a structured approach. That’s where data analytics frameworks come into play.
To tackle this challenge, data analytics frameworks have emerged as essential tools for navigating the complex world of data analysis. These frameworks provide a structured approach to analyzing and interpreting data, offering a systematic way to uncover patterns, trends, and hidden correlations.
Data analytics frameworks come in various forms, each designed to address specific analytical objectives and challenges. From traditional frameworks like descriptive and diagnostic analytics to more advanced approaches like predictive and prescriptive analytics, there is a wide range of options available to organizations seeking to harness the full potential of their data.
In this article, we will dive into the fascinating realm of data analytics frameworks, exploring the different types and understanding their unique characteristics and applications. Whether you are a data professional, business executive, or simply curious about the world of data analytics, this exploration will equip you with valuable insights into the diverse landscape of frameworks and help you navigate the ever-evolving field of data analysis.
What is Data Analytics Framework?
A data analytics framework is a structured approach or methodology that guides the process of analyzing and interpreting data to derive meaningful insights and make informed decisions. It provides a systematic way to handle data, perform analysis, and extract valuable information from the vast amount of data generated by organizations. Typically, a framework consists of a set of tools, techniques, and best practices that guide the entire data analysis process. The framework serves as a roadmap, ensuring that organizations follow a consistent and efficient process in their data analysis efforts.
It’s important to note that data analytics frameworks are not static and can evolve over time. As technology advances and new analytical techniques emerge, frameworks need to adapt to incorporate these developments. Organizations should continually evaluate and update their frameworks to stay at the forefront of data analytics capabilities.
Types of Data Analytics Frameworks
There are various types of data analytics frameworks, each with its own focus and objectives. Some commonly used frameworks include descriptive analytics, diagnostic analytics, predictive analytics, prescriptive analytics and cognitive analytics. Let’s explore each of these frameworks in more detail:
1. Descriptive Analytics
Descriptive analytics is a branch of data analytics that focuses on summarizing historical data to gain insights into past events or phenomena. It involves organizing and presenting data in a meaningful way through visualization techniques, such as charts, graphs, and dashboards. Descriptive analytics aims to provide a clear and concise snapshot of what has happened, allowing organizations to understand patterns, trends, and key metrics. By analyzing descriptive analytics, businesses can gain valuable insights into their past performance, customer behavior, and market trends, which can inform decision-making and help drive future strategies.
2. Diagnostic Analytics:
Diagnostic analytics is a form of data analytics that delves deeper into understanding the root causes and reasons behind specific events or outcomes. It goes beyond descriptive analytics by investigating the relationships between variables to uncover insights and explanations. Diagnostic analytics involves conducting exploratory analysis and applying statistical techniques to identify patterns, correlations, and anomalies within the data. By analyzing diagnostic analytics, organizations can gain a comprehensive understanding of why certain events occurred, enabling them to address underlying issues, optimize processes, and make informed decisions to prevent similar occurrences in the future.
3. Predictive Analytics:
Predictive analytics is a field within data analytics that employs historical data and statistical modeling methods to predict future outcomes or trends. Its objective is to make well-informed forecasts and estimations based on the analysis of patterns, correlations, and connections present in the data. By utilizing a range of statistical and machine learning algorithms, predictive analytics creates predictive models that enable organizations to anticipate customer behavior, market trends, demand patterns, and other important factors. This capability empowers businesses to proactively make decisions, optimize the allocation of resources, manage risks effectively, and gain a competitive edge by leveraging data-derived insights to shape their future strategies and actions.
4. Prescriptive Analytics:
Prescriptive analytics is an advanced field in data analytics that employs historical data, mathematical models, optimization algorithms, and simulation methods to offer guidance on the best actions or decisions to attain desired outcomes. Unlike descriptive and predictive analytics, which concentrate on understanding past occurrences and forecasting future trends, prescriptive analytics takes an additional step by proposing precise courses of action. By considering various limitations, goals, and potential situations, prescriptive analytics enables organizations to make data-informed choices that maximize the achievement of desired results. It supports businesses to enhance operational efficiency, allocate resources effectively, manage risks, and make well-informed decisions that drive improved performance and a competitive edge.
5. Cognitive Analytics:
Cognitive analytics refers to the application of advanced technologies and techniques that enable systems and machines to mimic human cognitive abilities, such as perception, learning, reasoning, and problem-solving. It combines elements of artificial intelligence, machine learning, natural language processing, and other cognitive computing technologies to analyze and interpret complex data sets. Cognitive analytics goes beyond traditional data analysis by incorporating context, understanding, and inference capabilities to derive deeper insights and make more informed decisions. It enables organizations to unveil patterns, trends, and relationships within large volumes of structured and unstructured data, leading to improved decision-making, enhanced customer experiences, and the discovery of valuable business opportunities.
These five types of analytics frameworks are not mutually exclusive and can often be combined to create more comprehensive analysis approaches. Organizations can select and tailor a framework based on their specific goals, data availability, and analytical capabilities.
In addition to these core frameworks, there are other considerations within a data analytics framework, such as data collection, data preprocessing, data exploration, and modeling techniques. These elements ensure that the data is appropriately prepared, outliers and missing values are addressed, and the most suitable analytical methods are applied.
Data Analytics Framework Steps
Data analytics frameworks typically comprises several steps to guide the process of analyzing data and extracting valuable insights. Here is a breakdown of the general steps involved in utilizing data analytics frameworks:
1. Define objectives
Clearly define the objectives and goals of your analysis. It is crucial to have a clear understanding of what you aim to achieve and how the insights derived from the analysis will contribute to your business objectives. Identify the specific questions you want to answer or the problems you want to solve through data analysis. This step is essential as it provides a clear direction for the entire analytics process.
2. Data collection:
Gather the relevant data needed for your analysis. This may contain accessing data from various sources such as databases, spreadsheets, or APIs. Ensure that the data is accurate, complete, and representative of the problem or question you are addressing.
3. Data preprocessing
Clean and prepare the data for analysis. This step consists of handling missing values, removing outliers, normalizing data, and transforming variables as necessary. Data preprocessing ensures that the data is in a proper format for analysis and helps mitigate any potential biases or errors.
4. Exploratory data analysis:
Conduct exploratory data analysis to gain initial insights and understand the characteristics of the data. This step involves visualizing the data using charts, graphs, and statistical summaries to identify patterns, trends, and relationships within the dataset.
5. Select appropriate techniques
Choose the appropriate data analysis techniques based on your objectives and the nature of your data. This may include statistical methods, machine learning algorithms, or data mining techniques. Select techniques that are suitable for the type of analysis you want to perform, such as descriptive, diagnostic, predictive, or prescriptive analytics.
6. Apply the chosen framework
Apply the selected data analytics framework, following the specific steps and procedures outlined within the framework. This may cover performing statistical tests, building predictive models, conducting hypothesis testing, or applying optimization algorithms, depending on the chosen framework.
7. Interpret and analyze results
Analyze the results obtained from your data analysis. Interpret the findings in the context of your objectives and consider their implications. Identify significant insights, patterns, or trends that provide meaningful information to address your initial questions or solve the problem at hand.
8. Communicate and visualize findings
Present the results of your analysis in a clear and understandable manner. Use visualizations, reports, and dashboards to effectively communicate the insights gained from the analysis. Tailor your communication to the intended audience, ensuring that the findings are accessible and actionable.
9. Evaluate and iterate
Evaluate the effectiveness of your data analytics framework and the insights derived from it. Seek feedback from stakeholders and domain experts to validate the results and identify areas for improvement. Use this feedback to refine your approach and iterate on the analysis process for future iterations.
10. Implement decisions and monitor outcomes:
Utilize the insights gained from the data analytics framework to make data-driven decisions and take appropriate actions. Monitor the outcomes of these decisions and assess their impact on the desired objectives. This step helps in measuring the effectiveness of the data analytics framework and its contribution to achieving business goals.
Remember that the specific steps and their order may vary depending on the data analytics strategy framework being used, the nature of the data, and the objectives of the analysis. Adapt the steps to fit your specific requirements and continuously refine your approach based on feedback and new insights.
Tips to Utilize Data Analytics Frameworks
To effectively utilize data analytics frameworks and harness the power of data, consider the following comprehensive tips:
1. Clearly define objectives
Begin by clearly articulating the goals and objectives of your data analysis. It is crucial to have a clear understanding of what you aim to achieve and how the insights derived from the analysis will contribute to your business objectives. Clearly defined objectives will help guide the entire data analytics process and ensure alignment with your organization’s strategic priorities.
2. Choose the right framework
Selecting the appropriate data analytics framework is key to achieving accurate and relevant insights. Consider the specific type of analysis required for your objectives. Whether it is descriptive analytics, diagnostic analytics, predictive analytics, or prescriptive analytics, each framework offers distinct approaches and methodologies to address different analytical needs. Familiarize yourself with the characteristics and applications of each framework to make an informed choice.
3. Gather relevant and quality data
Data is the foundation of any successful data analytics initiative. Ensure that you have access to accurate, reliable, and comprehensive data that is relevant to your analysis objectives. This may involve collecting data from various sources, cleaning and transforming it to ensure data quality, and organizing it in a structured format for analysis. Remember, the accuracy and quality of your insights depend heavily on the quality of the underlying data.
4. Validate and iterate
Data analysis is an iterative process. Continuously validate your analysis results and refine your framework as necessary based on feedback and new insights. Validate your findings by comparing them with real-world observations, conducting hypothesis testing, or seeking input from domain experts. Incorporate feedback from stakeholders to improve the effectiveness and relevance of your data analytics framework over time.
5. Incorporate feedback and continuous improvement
Actively seek feedback from stakeholders and incorporate their input to improve the effectiveness and impact of your data analytics framework. Regularly evaluate the performance of your framework, identify areas for improvement, and stay responsive to changing business needs and technological advancements. Embrace a culture of continuous improvement to ensure that your data analytics framework evolves along with your organization’s needs.
6. Stay updated:
The field of data analytics is rapidly evolving, with new techniques, methodologies, and technologies emerging regularly. Stay abreast of the latest developments by attending industry conferences, participating in training programs, and following reputable sources of information. Embrace new tools and technologies that can enhance your data analytics capabilities, such as advanced analytics software, cloud-based platforms, or artificial intelligence applications. Staying updated will help you leverage the latest advancements and maintain a competitive edge in the data-driven landscape.
By following these comprehensive tips, organizations can maximize the value derived from data analytics frameworks and drive data-informed decision-making processes for improved business outcomes.
In conclusion, a data analytics framework is a structured approach that enables organizations to extract meaningful insights from data. It provides a systematic process for handling data, performing analysis, and deriving actionable information. By leveraging different types of frameworks and techniques, organizations can harness the power of data to make informed decisions, optimize operations, and drive business success in today’s data-driven world.
If you have any questions or need assistance, please don’t hesitate to reach out to us. We are pleased to have been selected as the preferred destination for providing you with the solutions you seek.
More From Blog
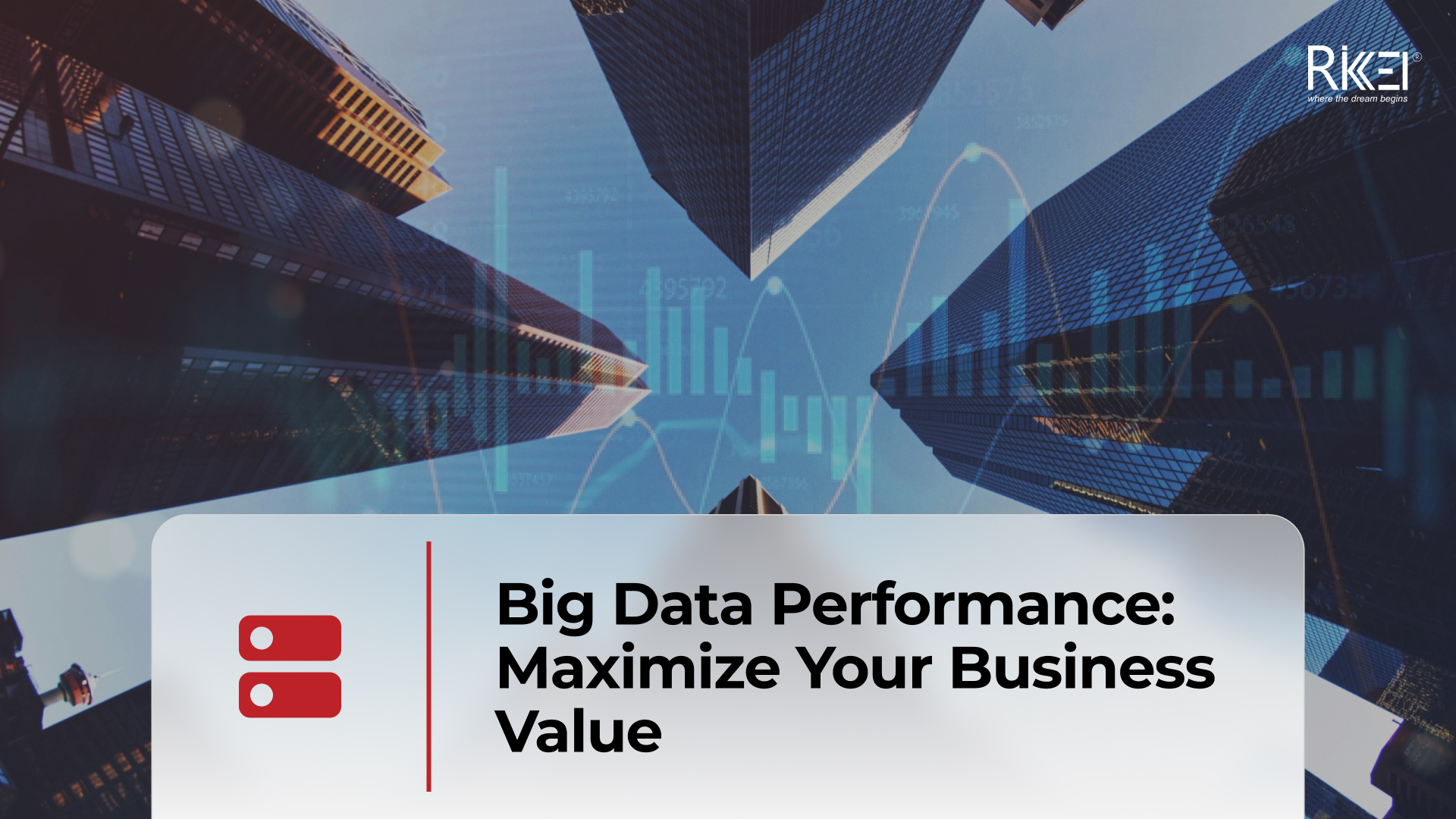
April 4, 2024
Big Data Performance: Maximize Your Business Value
In today’s data-driven world, organizations are constantly generating and collecting immense amounts of data to understand their customers more deeply. This data, often referred to as “big data,” holds immense potential for organizations to seek opportunities and overcome challenges. But accessing and analyzing big data isn’t enough to have proper strategies; organizations must pay attention to […]
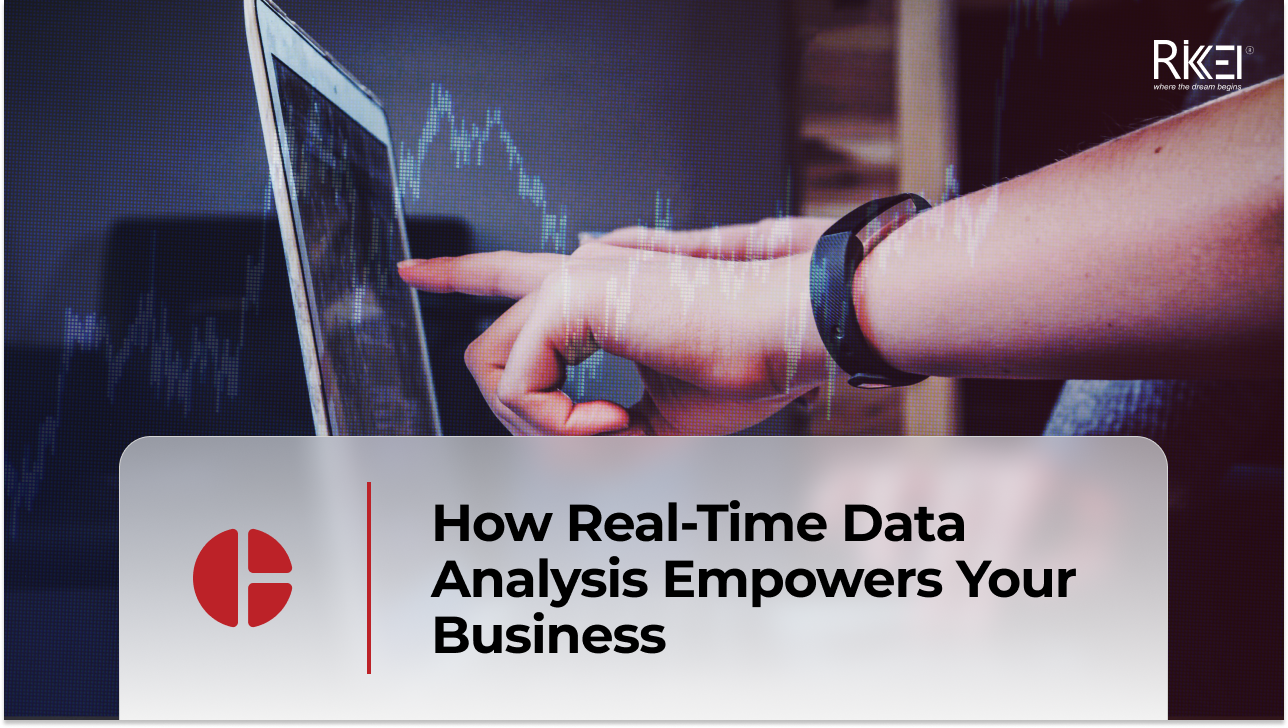
April 4, 2024
How Real-Time Data Analysis Empowers Your Business
In today’s fast-paced business landscape, the ability to quickly make data-driven decisions has become a key differentiator for success. Real-time data analysis, the process of analyzing data as soon as it’s generated, has emerged as a powerful tool to empower business across industries. By leveraging real-time data analysis, organizations can gain timely and actionable insights, […]
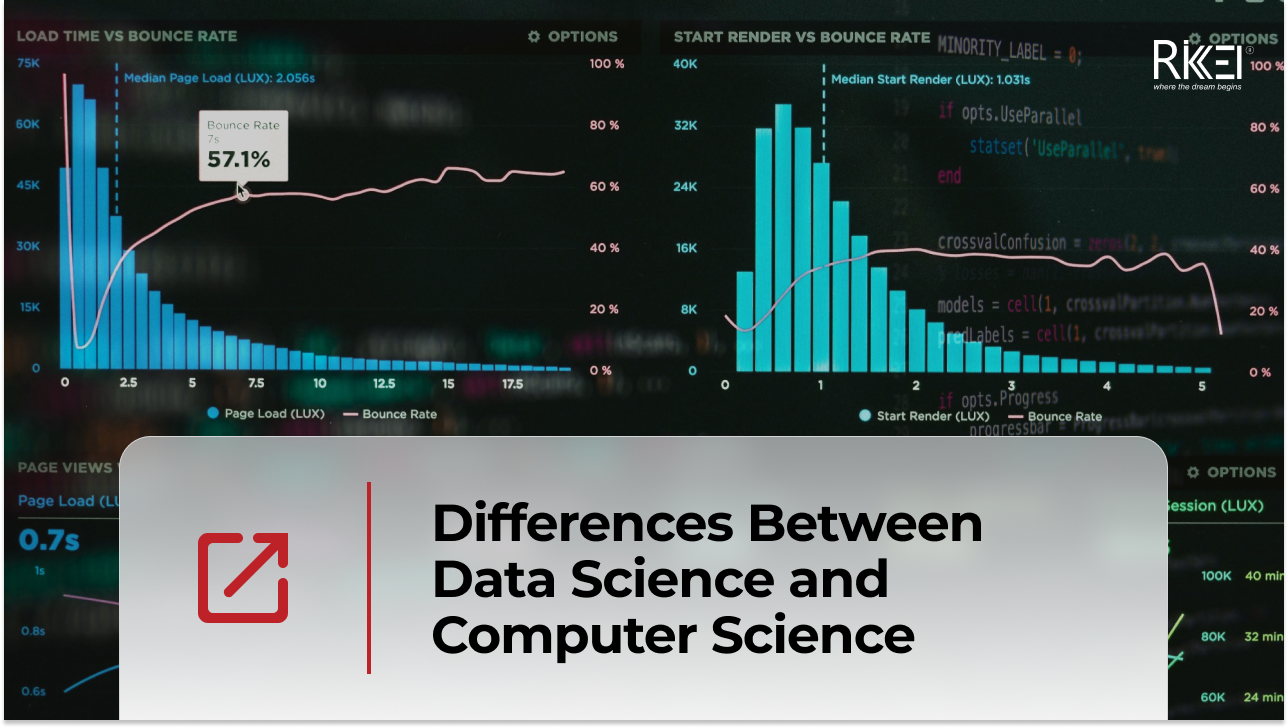
April 4, 2024
Differences Between Data Science and Computer Science
Data Science and Computer Science are distinct fields overlapping in certain areas but have different focuses and objectives. The article below will help you clearly understand the differences and the close connection between the two fields. What is Data Science? Data Science is an interdisciplinary field that combines scientific methods, processes, algorithms, and systems to […]
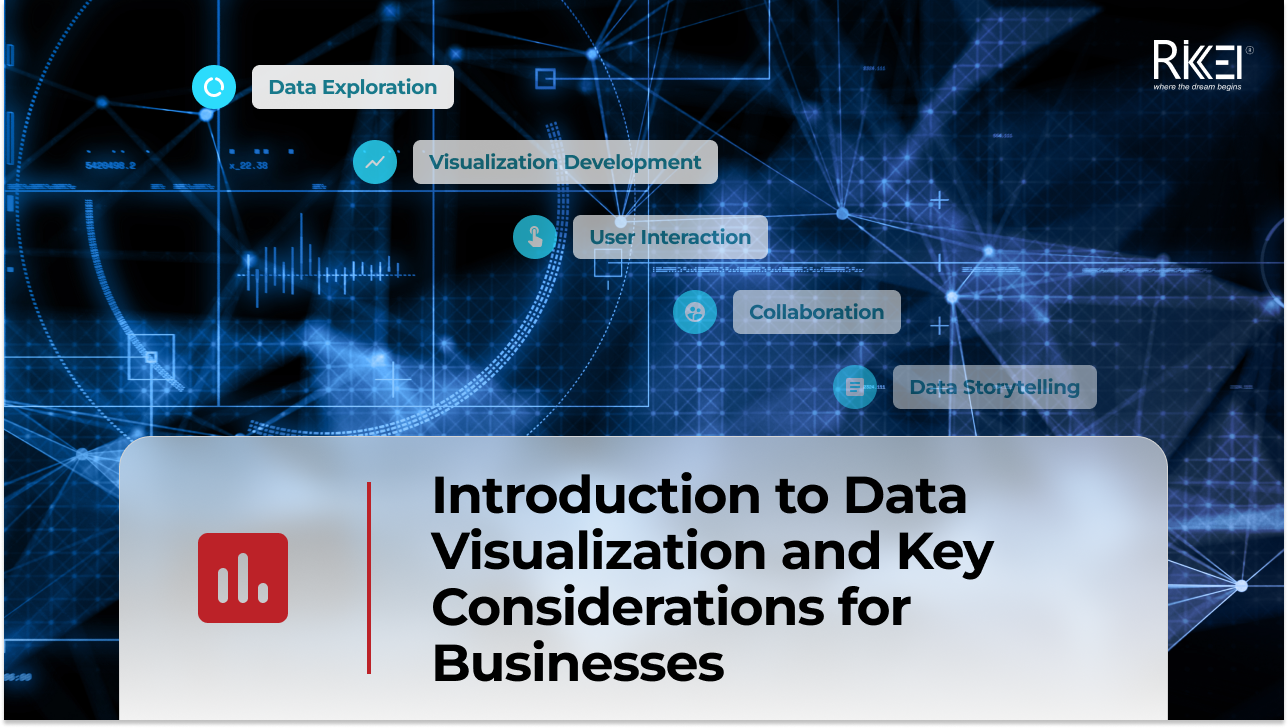
March 28, 2024
Introduction to Data Visualization and Key Considerations for Businesses
In your opinion, what is data visualization? Your main goal is to communicate your recommendations engagingly and effectively, right? To achieve this, let’s immediately explore a method that can represent information with images. What is Data Visualization? Define data visualization and their roles in organizations First, you need to find the answer to the question: […]
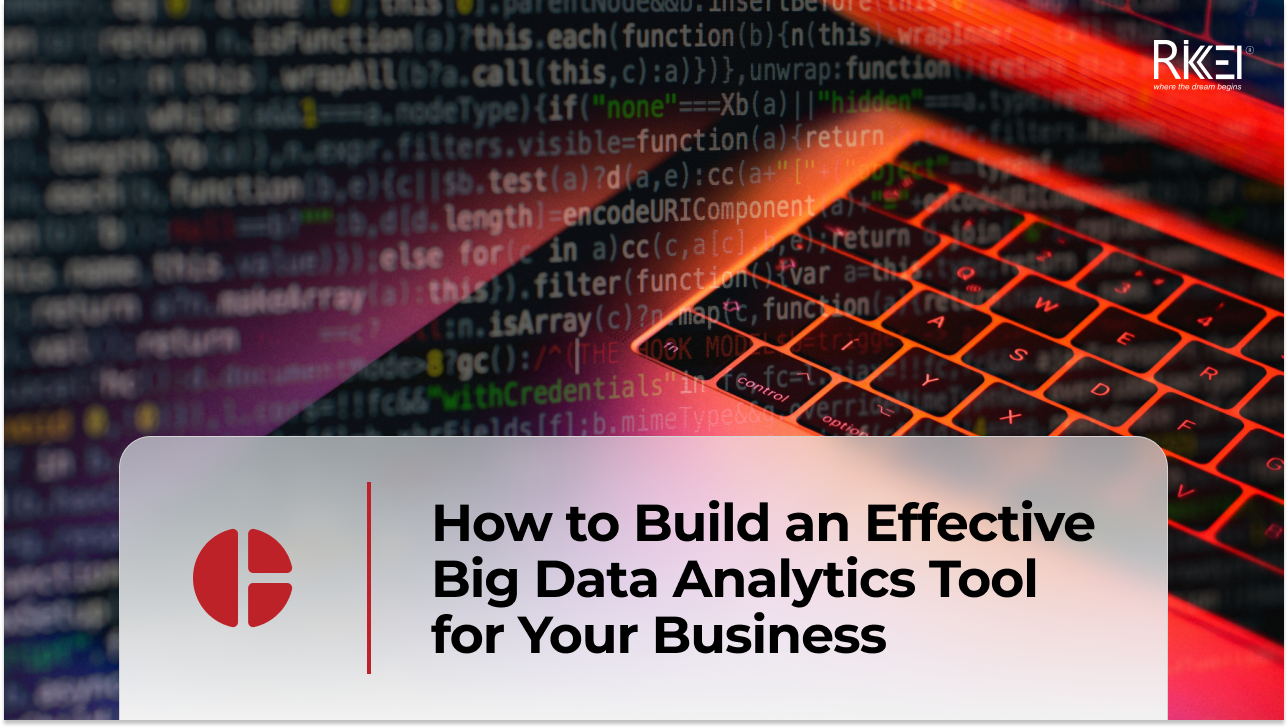
March 21, 2024
How to Build an Effective Big Data Analytics Tool for Your Business
Building an analytics tool for a business brings several significant benefits, especially in today’s business environment where data is becoming larger and more complex. So how to build an effective analysis tool for businesses, follow the article below! Assessing Business Needs Assessing business needs involves understanding the requirements, goals, and challenges of a business or […]
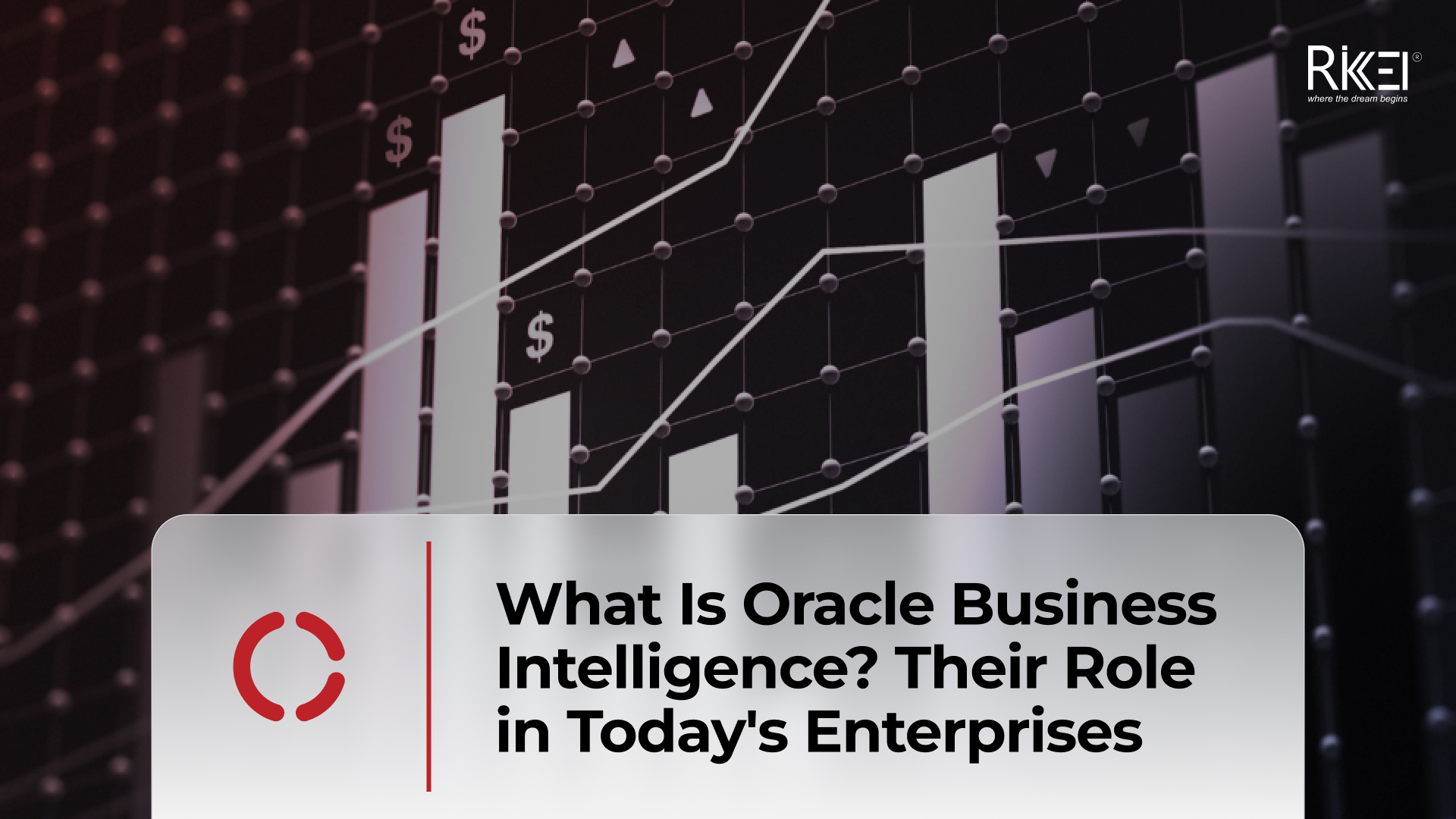
March 14, 2024
What Is Oracle Business Intelligence? Their Role in Today’s Enterprises
Oracle Business Intelligence (BI) refers to a suite of tools, technologies, and applications designed to help organizations collect, analyze and present business data. The primary goal of Oracle BI is to provide actionable insights to support decision-making within an organization. Oracle BI encompasses a range of products that enable users to gather, process and visualize […]