The Power of Big Data Application: Unlocking Insights and Opportunities
Contents
Big Data Application has revolutionized industries by unlocking insights that were previously hidden in the noise of vast datasets. Indeed, data plays an important role in our lives, enhancing efficiency and productivity in almost every field.
How to Use Big Data Application?
Data analytics empowers businesses in various sectors to address challenges in their operations. Some key benefits include: time savings, cost reductions, innovative product development, and enhanced customer attraction.
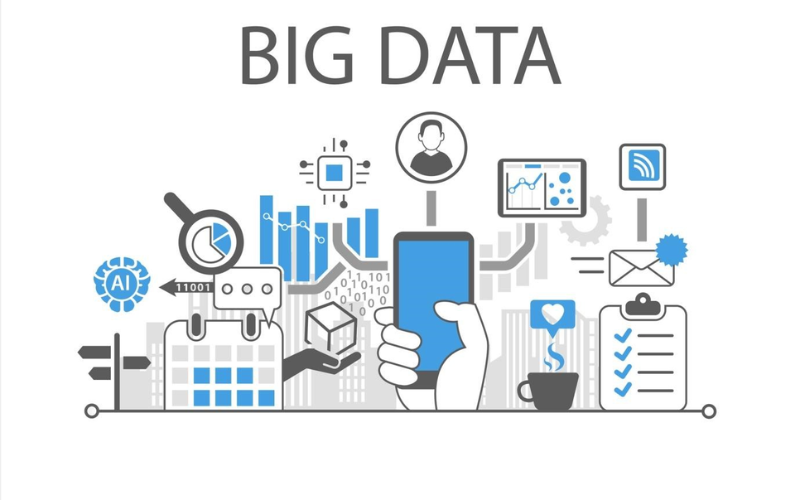
Here are some ideas to harness the power of big data for different industries and sectors:
Healthcare
When leveraged effectively in healthcare, big data can elevate patient care, reduce healthcare costs, and foster medical research.
- Utilize electronic health records and patient data to identify trends and patterns in medical history, diagnoses, treatments, and outcomes. This strategy enhances personalized patient care, enables early disease detection, and ensures treatment optimization.
- Forecast disease outbreaks by monitoring and analyzing data from various sources, including clinical records, social media, and environmental data. Early detection facilitates timely intervention and resource allocation.
- Examine genetic, clinical trial, and chemical data to swiftly identify potential drug candidates. This approach accelerates the drug discovery process and curtails costs.
- Spot healthcare fraud and abuse by analyzing insurance claims and billing data, thereby reducing fraudulent claims and healthcare costs.
- Streamline resource allocation, including staff scheduling, bed management, and equipment utilization, to boost hospital efficiency and reduce wait times.
- Leverage big data to improve public health outcomes by identifying at-risk groups, tracking disease prevalence, and crafting preventive health programs.
- Assist radiologists in diagnosing diseases and detecting abnormalities more accurately through medical imaging data (e.g., MRI, CT scans).
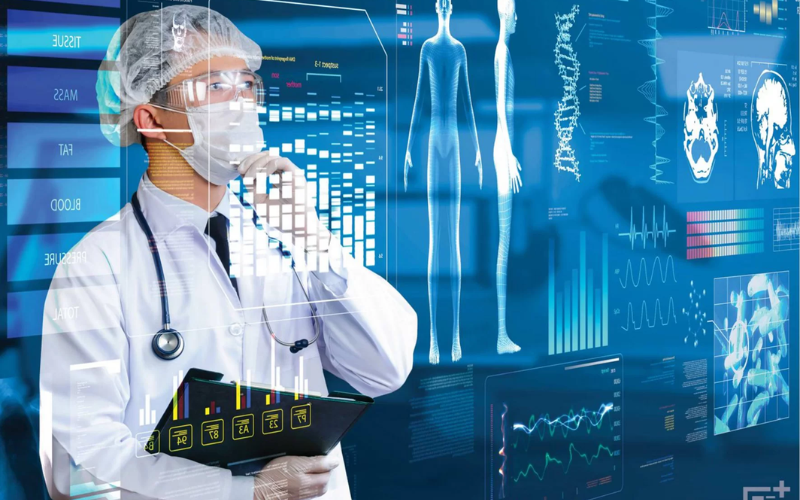
Retail
Harnessing big data in the retail industry enables businesses to improve customer experiences, streamline operations, and bolster profitability.
Ways to Leverage Big Data Application in the Retail Industry:
- Segment customers based on their purchase history, preferences, and demographics for targeted marketing and personalized product recommendations.
- Adopt demand forecasting models that analyze historical data and market trends to ensure optimal inventory levels.
- Set competitive prices using pricing optimization algorithms based on real-time market conditions, competitor pricing, and customer behavior.
- Boost supply chain efficiency by tracking product movement from suppliers to stores, predicting delivery times, optimizing routes, and strengthening vendor relationships.
- Craft targeted marketing campaigns using customer segmentation and personalized recommendations while monitoring campaign effectiveness.
- Refine store layouts, staffing, and product placements based on customer traffic patterns and buying behavior to elevate the in-store shopping experience.
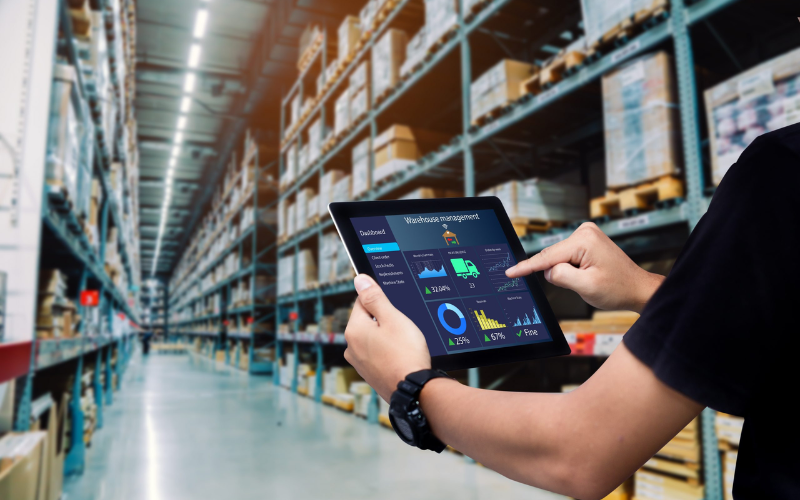
Finance
The finance industry’s heavy reliance on data makes big data analytics vital for risk management, investment strategies, and customer service. These applications help financial institutions remain competitive, compliant, and adaptable to changing market conditions.
- Evaluate credit risk, market risk, and operational risk by analyzing large datasets to predict defaults, fraud, and market fluctuations.
- Develop trading algorithms that analyze market data in real-time, executing trades swiftly.
- Gather insights from customer data, including transaction history and online behavior, to personalize marketing, recommend products, and bolster customer retention.
- Adopt big data solutions for AML (Anti-Money Laundering) compliance, analyzing vast transaction data to detect suspicious activities.
- Incorporate non-traditional data sources, such as social media activity, into credit scoring models for more accurate credit risk assessment.
- Forecast market trends, asset prices, and economic indicators to guide investment strategies.
- Optimize investment portfolios based on real-time market data and portfolio performance.
- Identify patterns in historical market data to enhance trading strategies.
Manufacturing
Handling big data in manufacturing leads to increased efficiency, improved product quality, and enhanced competitiveness in a rapidly evolving industry.
- Utilize machine and equipment data, such as temperature, vibration, and performance metrics, to predict when maintenance is needed. This approach minimizes unplanned downtime and reduces maintenance costs.
- Spot defects and anomalies in real-time. Big data can help improve product quality by flagging defective items early in the manufacturing process.
- Examine energy consumption data to identify opportunities for energy savings. By adjusting equipment settings and scheduling operations during off-peak hours, energy usage can be optimized.
- Leverage historical sales data, market trends, and external factors to predict future demand for products. This insight aids in production planning and inventory management.
- Strategize to optimize inventory levels and reduce carrying costs. By tracking and adjusting inventory in real-time based on demand fluctuations, inventory management becomes more efficient.
- Oversee worker safety and productivity using sensors and wearable devices. By analyzing this data, a safe working environment is ensured, and opportunities for efficiency improvements are identified.
- Customize products to individual customer preferences without compromising cost-effectiveness. This approach allows for more personalized and flexible manufacturing.
Advice for Big Data Application
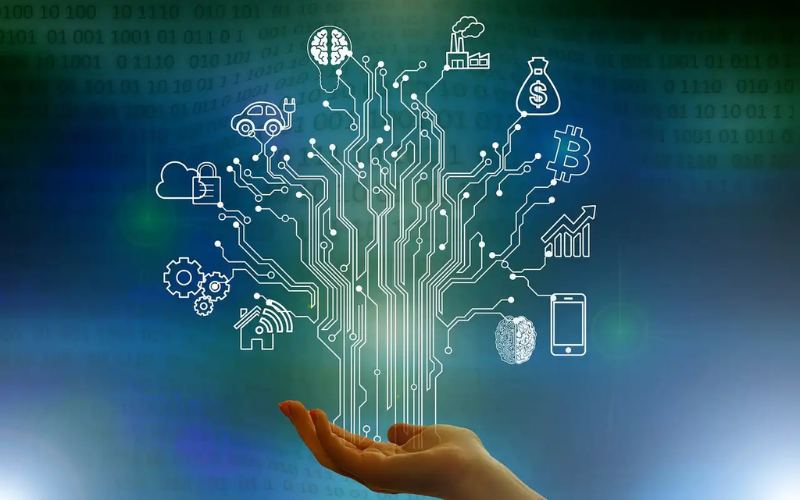
Here are some essential pieces of advice for successful Big Data Application:
Start Small
Starting small is a prudent approach to implementing big data effectively, as it allows you to gain experience, validate concepts, and gradually expand your efforts as you learn.
Begin by identifying a specific use case and setting clear goals. Collect relevant data, focusing on taking advantage of available data. The choice of a data analysis tool is also very important; today, many tools cater to small-scale projects.
Monitor costs, especially when starting small. Minimize unnecessary expenditures and leverage existing resources whenever possible. As you achieve success and gain confidence in your big data capabilities, consider scaling up your initiatives. Expand to more complex use cases and larger datasets.
Choose the Right Tools
Selecting the right tools is crucial for the effective application of big data in your projects. To determine whether a tool is suitable for your organization, consider the following tips:
- Ensure the tools align with your requirements and understand what you aim to accomplish and the specific challenges you want to address.
- Look for flexibility and customization. Depending on the project, there may be adjustment requirements to meet business needs.
- Assess the scalability and performance capabilities of the tools. Big data often involves large datasets, and the tools should be able to process and analyze data efficiently as it grows.
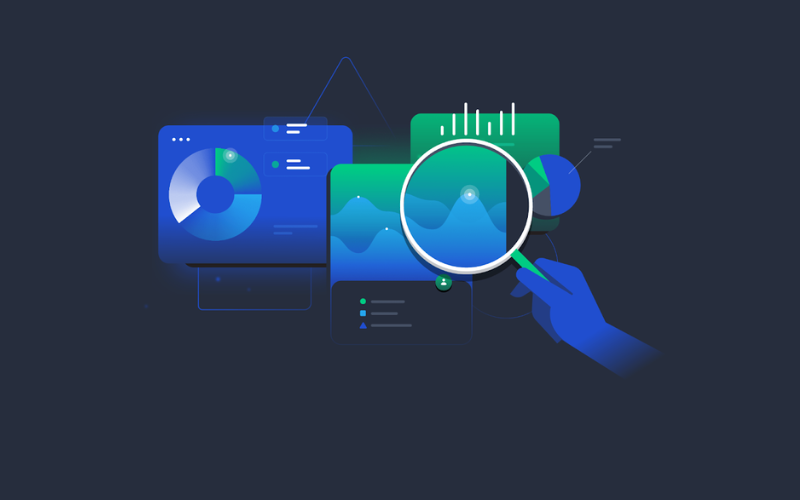
- Verify that the chosen tools can integrate seamlessly with your existing technology stack. Compatibility with databases, data storage systems, and other software is essential.
- For commercial tools, check the level of support and maintenance provided by the vendor. If support is slow, reconsider your choice.
- Evaluate the cost of tools against your budget. Prioritize tools with robust security features and data privacy capabilities.
Define Clear Goals and Metrics
Defining clear goals and metrics is a fundamental step in ensuring the effective application of big data in your projects. Here are some essential pieces of advice for setting clear goals and metrics in your Big Data Application:
- Understand your business objectives: Before diving into big data projects, ensure a deep understanding of your organization’s overarching business objectives. Your goals should align with and support these objectives.
- Engage key stakeholders from different departments: including IT, marketing, operations, and finance, to gain a holistic view of organizational needs and objectives.
- Focus on specific use cases or challenges: Trying to address too many issues at once can dilute your efforts and make goals less clear.
- Set SMART (specific, measurable, achievable, relevant, and time-bound) goals: Use quantitative metrics whenever possible to track progress and success.
- Clearly outline what success looks like for each goal: For example, if you aim to improve customer retention, specify the percentage increase you want to achieve within a defined timeframe.
Emphasize Data Quality and Governance
Emphasizing data quality and governance is essential for effective Big Data Application.
- Define Data Governance Policies: Establish clear data governance policies and guidelines that define roles, responsibilities, and processes for managing and maintaining data throughout its lifecycle.
- Data Ownership and Stewardship: Assign data ownership and stewardship roles within your organization. Data owners are responsible for the quality and integrity of specific datasets.
- Data Security and Privacy: Ensure that data governance practices include robust data security and privacy measures. Adhere to relevant regulations to protect sensitive information.
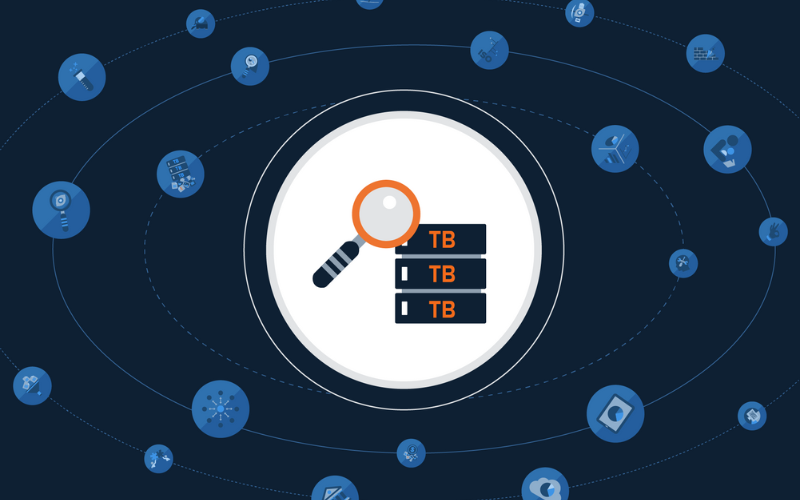
- Data Access Controls: Implement access controls and permissions to restrict data access to authorized individuals or roles. Apply the principle of least privilege.
- Data Quality Metrics: Develop data quality metrics and key performance indicators (KPIs) to measure the effectiveness of your data quality efforts.
- Data Governance Committee: Form a data governance committee with representatives from various departments to oversee data governance initiatives and resolve data-related issues.
Build a Skilled Team
Building a skilled big data team is an ongoing process that requires attention to both technical skills and soft skills. A well-rounded team with diverse expertise is essential for tackling the complex challenges of Big Data Applications effectively.
- Define the roles and responsibilities within your big data team and identify the specific skills and competencies required for each role.
- Recruitment and Hiring: When hiring team members, look for candidates with a strong background in data science, statistics, machine learning, or relevant technical disciplines. Seek individuals with a track record of successfully working on similar projects.
- Provide Training and Development: Invest in ongoing training and development programs for your team. The field of big data is continually evolving, and staying current is essential.
- Team Communication: Establish effective communication channels within the team to facilitate collaboration, share insights, and address challenges promptly.
- Ethical Considerations: Ensure that team members are aware of ethical considerations related to data, especially when handling sensitive or personal information.
- Celebrate Successes: Celebrate project successes and milestones as a team. Recognize and reward outstanding contributions.
- Long-Term Planning: Develop a long-term talent strategy to ensure that your team can continue to meet the evolving needs of your big data initiatives.
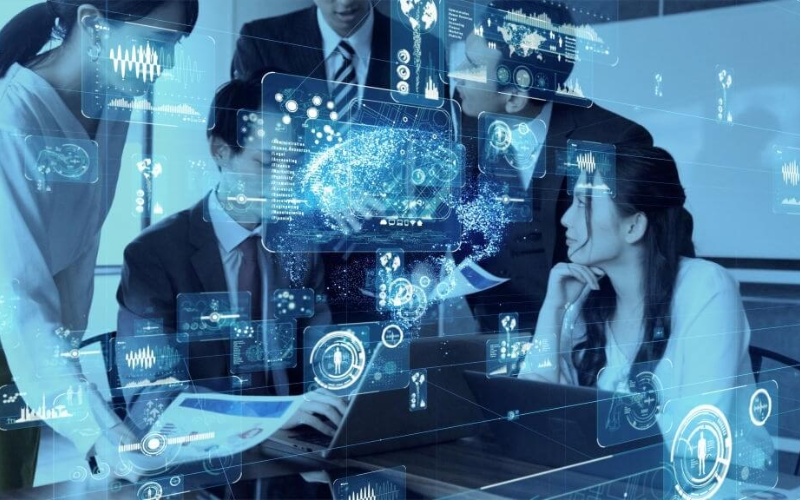
Choose a reliable service provider
Choosing a reliable service provider like Rikkeisoft for your Big Data Application can significantly impact the success of your projects. Why should you choose Rikkeisoft?
- As a top 5 enterprise software development company, we have many years of experience in the data and a highly specialized team.
- We guarantee a variety of services to suit your needs, including data analysis, data engineering, data visualization, machine learning, and more.
- We prioritize data security measures and adhere to relevant data privacy regulations to protect your sensitive information.
- We ensure that we can handle your data volume and growth requirements effectively.
- Our focus on governance and quality prioritizes data accuracy, completeness, and reliability.
- Our use of advanced and modern technology can integrate with your data sources.
- Rikkeisoft can accommodate custom solutions if necessary.
Conclusion
Remember that successful Big Data Application is an ongoing process. It involves continuous learning, adaptation, and a commitment to data-driven decision-making as a core part of your organization’s culture and strategy.
More From Blog
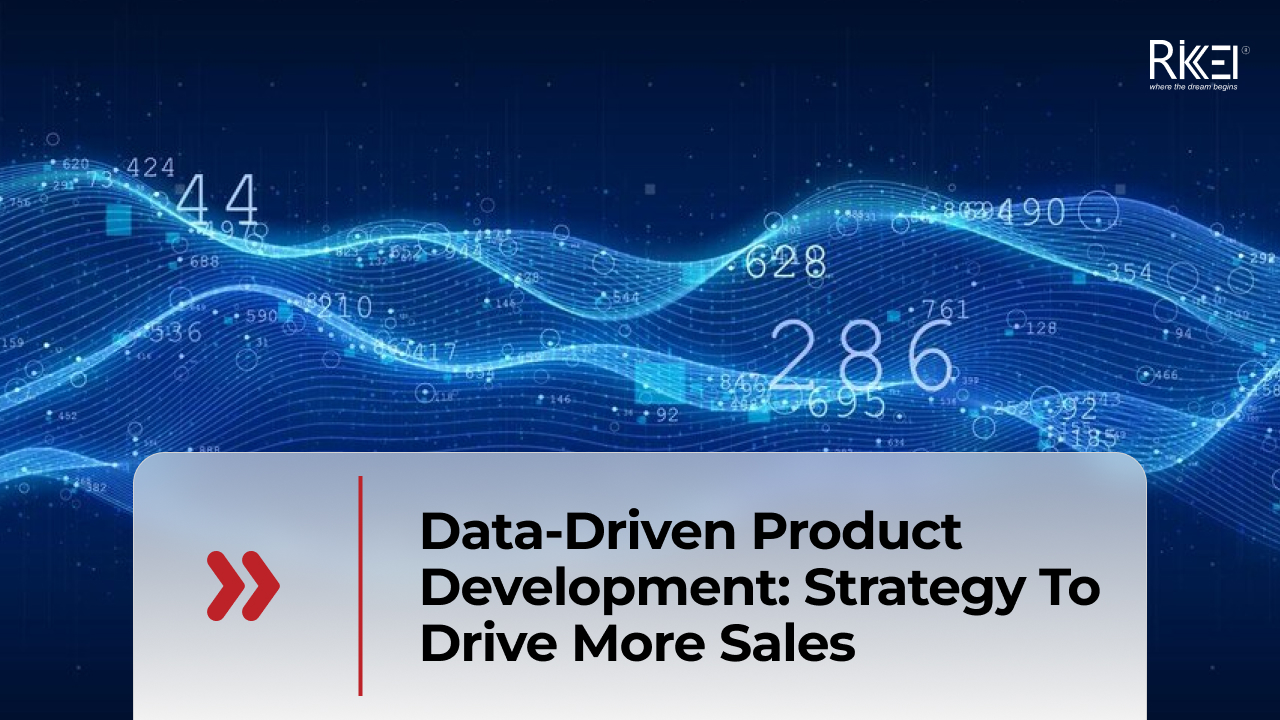
August 8, 2024
Data-Driven Product Development: Strategy To Drive More Sales
As a business owner, you want your products or services to be well-received upon launch. The most effective way to create a product that satisfies a broad range of customers is to gain insights into their needs and behaviors from the outset. The key lies in data-driven product development, a strategy that many companies have […]
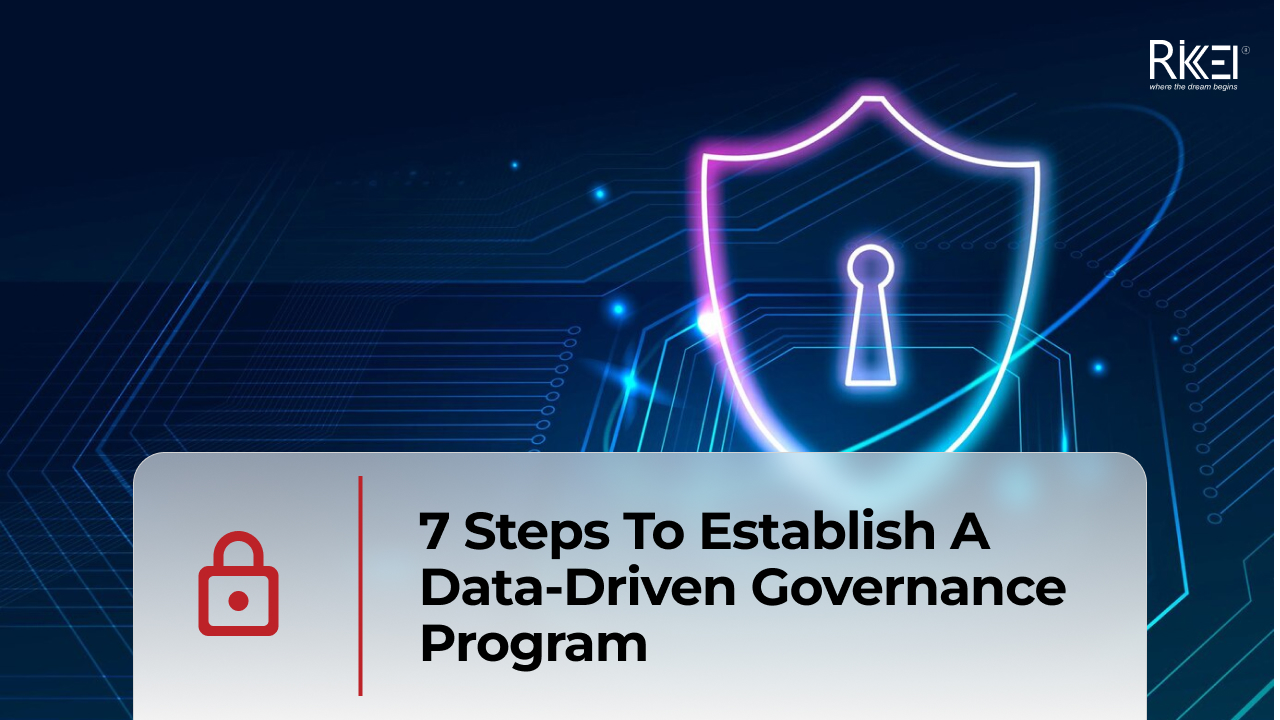
August 8, 2024
7 Steps To Establish A Data-Driven Governance Program
While data-driven approaches significantly benefit organizations in various ways, failure to govern the huge data sets will hurt your business even more. Effective data management also ensures data quality and security. That’s why there is an increasingly high demand for data-driven governance programs. Continue reading for a detailed guide! What Is Data-Driven Governance? Surprisingly, many […]
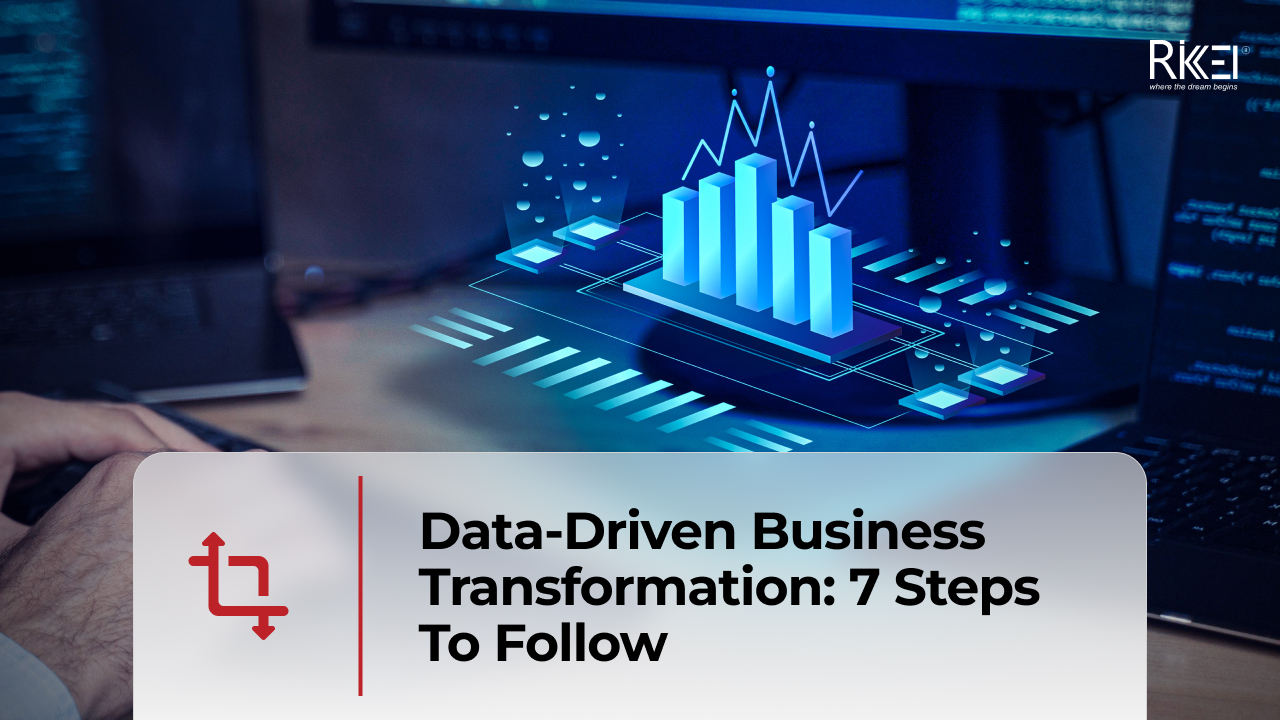
August 8, 2024
Data-Driven Business Transformation: 7 Steps To Follow
Data empowers businesses to make well-informed decisions in different departments, like marketing, human resources, finance, and more. As a business owner, you should also employ data-driven approaches to skyrocket productivity and efficiency. If you are still new to this concept, scroll down for an in-depth guide on data-driven business transformation. What Does A Data-Driven Business […]
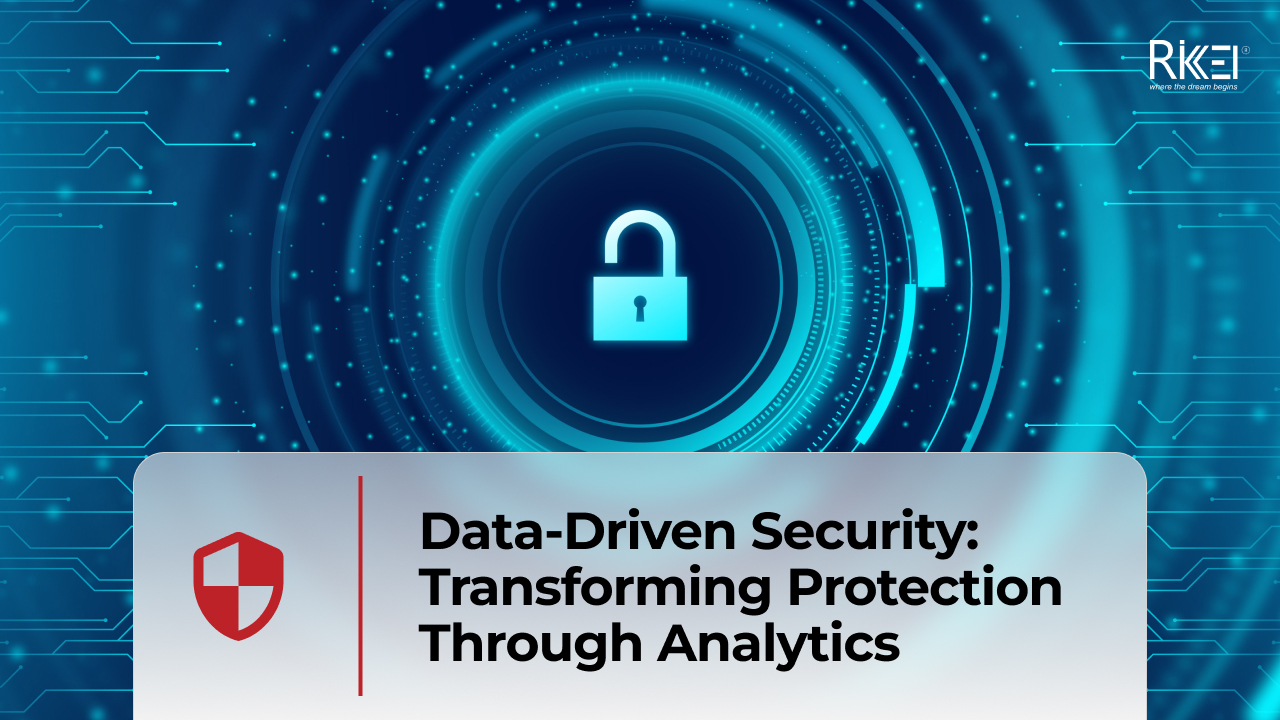
August 8, 2024
Data-Driven Security: Transforming Protection Through Analytics
Cybersecurity was once an afterthought for most organizations. But in today’s digital landscape, it has become mission-critical. With this transformation has also come a shift in how security decisions are made. Rather than relying solely on intuition and tradition, leading organizations are embracing data-driven strategies. By using metrics and insights around threats, vulnerabilities, and more, […]
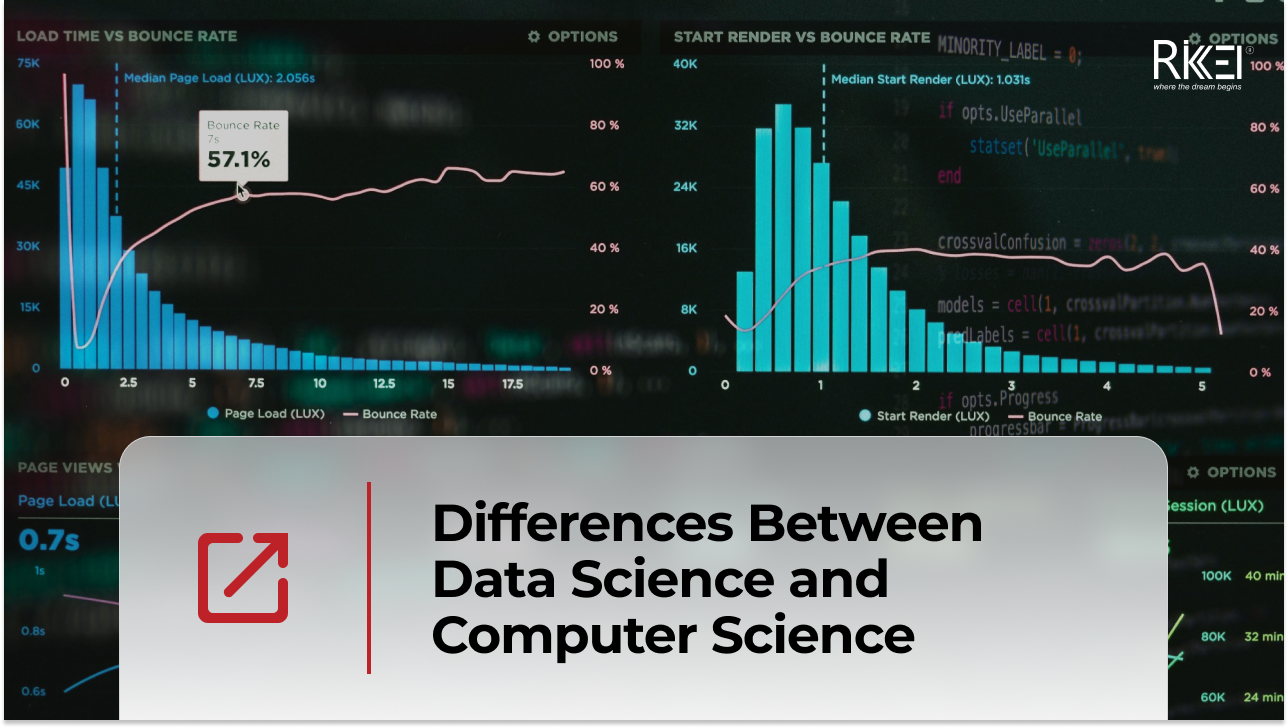
August 8, 2024
Differences Between Data Science and Computer Science
Data Science and Computer Science are distinct fields overlapping in certain areas but have different focuses and objectives. The article below will help you clearly understand the differences and the close connection between the two fields. What is Data Science? Data Science is an interdisciplinary field that combines scientific methods, processes, algorithms, and systems to […]
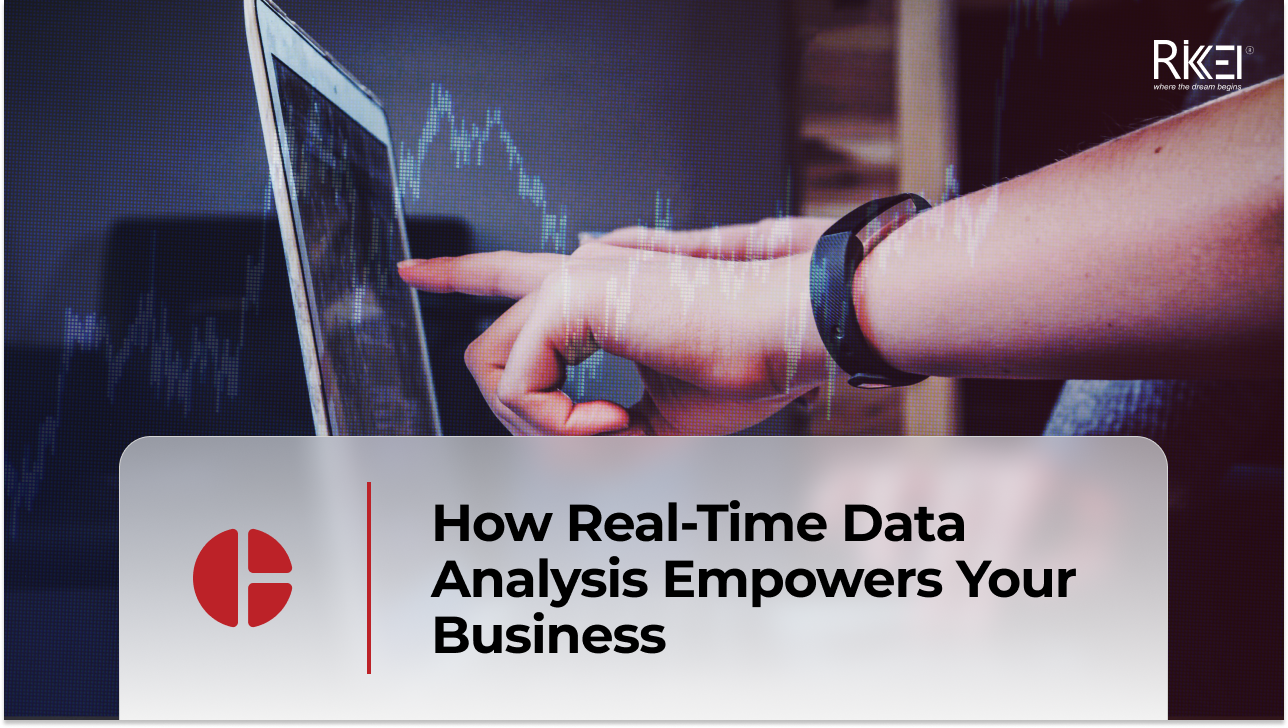
August 8, 2024
How Real-Time Data Analysis Empowers Your Business
In today’s fast-paced business landscape, the ability to quickly make data-driven decisions has become a key differentiator for success. Real-time data analysis, the process of analyzing data as soon as it’s generated, has emerged as a powerful tool to empower business across industries. By leveraging real-time data analysis, organizations can gain timely and actionable insights, […]